Why is there resistance to considering AI for manuscript evaluation? An interview with Alessandro Checco
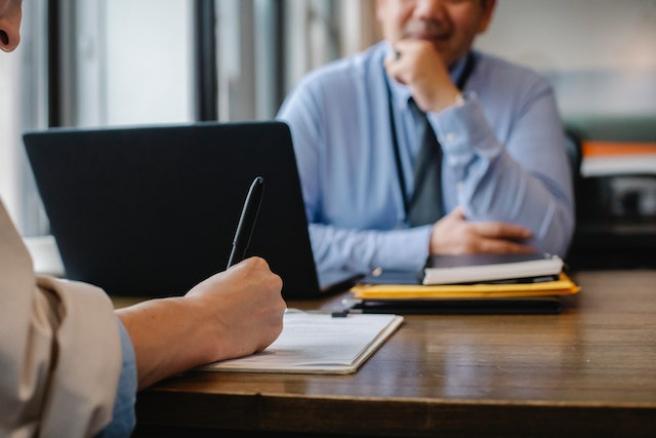
I am in an interesting conversation with Alessandro Checco on what role artificial intelligence tools and machine learning can play in the peer review process and whether AI can aid research integrity.
Alessandro Checco is Assistant Professor at computer science department, University of Rome La Sapienza (https://alessandrochecco.github.io/). He’s a mathematical engineer and has a PhD in mathematics of future wireless networks from Hamilton Institute, National University of Ireland Maynooth. Alessandro has published several papers, one of which centers around AI-assisted peer review (https://www.nature.com/articles/s41599-020-00703-8). His main research interests are human computation, recommender systems, information retrieval, data privacy, societal and economic analysis of digital labour, and algorithmic bias.
An increasing number of journals are embracing AI tools to support their peer review process. For the uninitiated, can you briefly explain in what ways are these tools being used currently?
While AI tools are not yet used directly to assess the quality of a manuscript, there are some tools that support the peer review process, such as tools for plagiarism detection, requirements and compliance checks, and reviewer-manuscript matching. Notable ones are:
- Automated tools used in the grant-review processes of the National Natural Science Foundation of China, to reduce bias and the load on the selection panels.
- Statcheck, software that assesses the consistency of authors’ statistics reporting.
- Online system to manage the grant application process, introduced in 2012 by the Canadian Institutes of Health Research (CIHR), removing the need for face-to-face meetings, to reduce reviewer fatigue and improve quality, fairness and transparency.
However, these tools are still in their infancy and some have had a brush with controversy. Some doubts have been expressed about the reliability of the Statcheck tool and the CIHR application system has received heavy criticism from some reviewers.
At the moment, one of the main limitations to using AI tools is the ability to reliably evaluate the quality of scientific content and the risk of introducing biases in the models used.
It is interesting that in the paper you’ve co-authored, it was found that the machine learning system you were experimenting with was able to predict the human peer review outcomes with remarkable accuracy. What are your inferences from this finding?
There are two possible explanations (or a mix of the two) as to why it was sufficient to use relatively superficial features such as word frequency distributions, readability scores, and formatting features to predict the quality of a paper.
One possible explanation is that if a paper lacks in presentation and reads badly, it is likely to be of lower quality in other ways too, making these more superficial features proxy useful metrics for quality evaluation. This is a heuristic method in deciding the overall quality of the paper.
As an extension, it would be reasonable to use AI to screen papers before the peer review process to desk reject papers based on these macroscopic features. This would save the time and effort of peer reviewers who would have had to otherwise review papers which were highly likely to be low quality.
Another explanation is that papers that score less well on these superficial features might create a “first impression bias” on the part of peer reviewers, who then are more inclined to reject papers based on this negative first impression derived from what are arguably relatively superficial problems. In other words, it’s possible that our AI was learning the “lazy” human behaviour of subconsciously looking for easy-to-recognise problems to quickly dismiss a paper. This could be caused by the pressure reviewers face to meet deadlines.
Reviewers may be unduly and subconsciously influenced by aspects such as formatting or grammatical issues which reflect in their judgements of more substantive issues in the submission. Examples of such issues in papers include the presence of typos, the presence of references to papers from regions under-represented in the scientific literature, or the use of methods that have been associated with rejected papers.
In that case, an AI tool which screens papers prior to peer review could be used to advise authors to rework their paper before it is sent for peer review. This is because it is likely that peer reviewers may reject the paper or at least be negatively influenced by the macroscopic features of the paper, which could be relatively easily corrected.
This might help authors for whom English is not a first language, for example, and whose work may likely be adversely affected by first impression bias.
At the moment we are not able to exactly quantify how much each of these factors affect the final outcome.
Can the use of AI tools bring objectivity to peer review, a process that to a large extent is considered subjective? In connection to this, are there any ethical concerns around using AI?
Even before considering AI, we should acknowledge that the peer review process is inherently subjective, because its rules are implemented by a group of people that decide what is interesting, what is impactful, what is rigorous. Thus, we should recognise the effect that the social contexts and hierarchies have on the fairness and equity of outcomes. In this scenario, objectivity risks to become a totem used to hide established power. For this reason, rather than only chasing impartiality and objectivity, it is important to strive towards transparency and representation of marginalised groups when building a system of quality evaluation. These issues can get amplified when using automated systems, especially if they are mistakenly considered objective or impartial.
From our experience, there is still a lot of resistance to considering AI for the cognitive, complex task of quality evaluation. But even for simpler tasks like the ones discussed before (e.g. plagiarism checks, reviewers allocation), the main reason for such resistance is the lack of transparency AI tools offer.
An author will not trust an automated review if there is no transparency on the rationale for the decision taken. This means that any tools developed to assist decision making in scholarly communication need to make what is going on under the bonnet as clear as possible. This is particularly the case since models are the result of a particular design path that has been selected following the values and goals of the designer. These values and goals will inevitably be “frozen into the code.”
Another reason for resistance to AI is the bias that can creep in when AI tools are used to assist a human reviewer: tools designed to assist reviewers can influence them in particular ways. Even using such tools to signal potentially problematic papers could affect the agency of reviewers by raising doubts in their minds about a paper’s quality. The way the model interprets the manuscript could influence the reviewer, potentially creating unintended biased outcomes.
All of these ethical concerns need to be considered carefully when AI tools are designed and deployed and when their role is determined in decision-making. Continued research in these areas would be crucial in ensuring that the role AI tools play in processes like peer review is a positive one.
In your opinion, can AI improve and safeguard quality controls in scientific research publication? If yes, how?
There isn’t direct evidence yet of real-world application of AI-assisted peer -review in the direct evaluation of the quality of a manuscript, but some developments indicate the ability of saving time in the reviewer-manuscript matching process; of course, the plagiarism detection tools are naturally easy to implement.
A lot of the tedious tasks that an editor has to carry out for each manuscript can now be automated, such as plagiarism detection, formal checks, and reviewer matching. This has a direct impact on saving time in the review process, allowing the allocation of precious human time to more important tasks.
I think there is still a lot of work to be done in this area. Natural language processing (NLP) tools can barely “understand” the meaning of single sentences or paragraphs at this point. Probably it’s too early to let them evaluate the quality of an entire manuscript with respect to originality, relevance, or rigour. But I would be happy to be contradicted!
Looking into the future, which aspects of machine learning systems or AI tools do you think require further research and development so that they can support research integrity better?
Firstly, my team and I are interested in exploring the behaviour of reviewers when using these AI-powered support tools. We intend in the future to carry out controlled experiments with academic reviewers to understand the biases introduced among reviewers by the AI signals.
Moreover, we would like to consider detailed reviewer feedback (rather than just the final score) when training our models. This is because the reviewer feedback and rebuttals that inform the final decision contain a great deal of useful information; thus, this could really improve the quality of our models.
From a technical point of view, it is necessary to work on bigger datasets to build models that can be more reliable. This is not easy because of the confidentiality of the review process.
Finally, we would like to see a more detailed study on the role of first impression bias and an understanding on how the human review process works when reviewers are under stress.
Thank you for speaking with us, Alessandro!