What is Regression Discontinuity Design? Applications for biomedical research
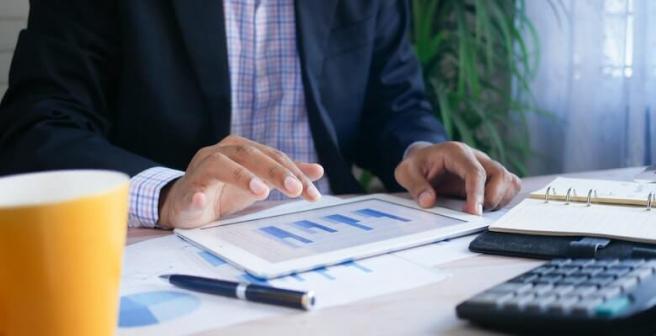
Regression Discontinuity Design (RDD) might sound like a complicated term, but don’t be intimidated! It’s actually a fairly straightforward and powerful method used in biomedical research to examine the effects of treatments, interventions, or policies. In this blog post, we’ll break down what RDD is, how it works, and explore some fascinating applications of RDD in answering pressing research questions.
What is Regression Discontinuity Design (RDD)?
At its core, RDD is a research design that allows scientists to evaluate the impact of an intervention when it is applied based on a specific cutoff or threshold. This means that subjects just above or below this threshold are treated differently, providing an opportunity to assess the intervention’s effectiveness.
How Does Regression Discontinuity Design (RDD) Work?
Imagine a simple scenario: a new drug is introduced to treat a certain medical condition, and doctors decide to prescribe it to patients only if their illness severity score exceeds a particular threshold. Patients just above this threshold receive the drug, while those just below it do not.
Let’s say doctors want to test a new blood pressure medication. They decide to prescribe it only to patients whose blood pressure is higher than 150/90 mm Hg. Patients with blood pressure just above this threshold get the medication, while those just below do not. RDD helps researchers compare the health outcomes of these two groups.
Advantages of Using Regression Discontinuity Design (RDD)
Causality Inference: RDD allows researchers to make causal inferences about the effects of an intervention, treatment, or policy. By comparing outcomes on either side of the threshold, it helps establish a cause-and-effect relationship.
Internal Validity: RDD minimizes selection bias because individuals just above and below the cutoff are, in theory, similar in all aspects except for the treatment or intervention they receive. This enhances the internal validity of the study. In the above example of a blood pressure lowering medication, using a conventional design could mean that some patients could have very high values, such as 180/110 mmHg, while others could have values fairly close to normal, such as 132/81 mmHg. Including these patients in the analysis could mean that we can’t get an accurate picture of whether the drug is truly efficacious for patients with hypertension.
Natural Experiment: RDD often arises from real-world situations where decisions are based on predetermined rules or thresholds. This provides a natural experiment setting, making the results more applicable to real-life scenarios.
Cost-Efficient: RDD can be a cost-effective research design because it leverages existing policies or criteria to create treatment and control groups without additional randomization efforts.
Disadvantages of Using Regression Discontinuity Design (RDD)
Cutoff Choice: Selecting the appropriate threshold or cutoff point is crucial in RDD. Choosing the wrong cutoff can lead to biased results or obscure the true treatment effect.
Generalizability: RDD results may not always generalize well to different populations or settings. The findings are specific to the chosen threshold and context, limiting external validity.
Ethical Concerns: In some cases, implementing RDD may involve withholding a potentially beneficial treatment or intervention from individuals just below the threshold. This can raise ethical concerns about fairness and equity.
Assumption of No Manipulation: RDD assumes that individuals cannot manipulate their assignment status around the threshold to receive the treatment. However, this assumption may not always hold in practice.
Small Sample Sizes: RDD studies often involve small sample sizes, particularly when the threshold is narrowly defined. This can limit the statistical power of the analysis and make it harder to detect meaningful effects.
Regression Discontinuity Jump: Sometimes, RDD studies may exhibit a sudden jump or discontinuity in outcomes at the threshold itself, which can complicate the interpretation of results.
Sensitivity to Model Specification: RDD results can be sensitive to the choice of statistical models used for analysis, which can lead to different conclusions depending on modeling decisions.
In summary, while RDD is a powerful tool for causal inference in certain situations, researchers must carefully consider its advantages and disadvantages when planning and interpreting studies using this design. The choice of cutoff, ethical considerations, and potential limitations should be weighed against the benefits of the design for each specific research question.
Applications of Regression Discontinuity Design (RDD) in Biomedical Research
Now, let’s explore some exciting applications of RDD in the field of biomedical research.
Assessing the Effectiveness of Vaccines
Biomedical researchers have used RDD to evaluate the impact of vaccination programs. For instance, they may set a cutoff age for vaccine eligibility and compare the health outcomes of individuals who just meet the cutoff (vaccinated) with those who just miss it (unvaccinated).
Suppose researchers want to study the effectiveness of the HPV vaccine. They may offer the vaccine to individuals up to the age of 26 and then compare the outcomes between those just below this age limit and those just above it using RDD. This helps determine how well the vaccine works in preventing HPV-related diseases.
On similar lines, Frio and França (2017) used RDD to show that HPV vaccination does not affect the age at which girls become sexually active nor risky sexual behavior, thus providing evidence to support HPV vaccination.
Evaluating the Efficacy of Interventions
RDD can also be used to assess the effects of medications, like in our blood pressure medication example. Researchers can set thresholds based on various factors, such as age, disease severity, or biomarker levels, to determine who receives the treatment. Take a look at how Odden et al. (2023) used RDD to estimate the effect of statins on myocardial infarction.
In cancer drug trials, RDD can be applied by offering an experimental drug to patients with certain tumor characteristics (e.g., tumor size or genetic markers) just above a predefined threshold. Comparing their outcomes with patients just below the threshold helps determine the drug’s effectiveness.
Investigating Healthcare Policies
RDD is handy for studying the effects of healthcare policies. Researchers can use specific criteria or cutoffs to allocate resources, like Medicaid benefits, and then analyze the impact on health outcomes.
In the case of Medicaid expansion, researchers can use RDD by comparing health outcomes between low-income individuals who qualify for Medicaid (just above a certain income threshold) and those who do not (just below the threshold). This helps policymakers understand the benefits of expanding healthcare coverage.
Further reading: Boon et al. (2021) provide a comprehensive review of how RDD has been used in over 5 decades of health research, highlighting shortcomings in study quality and reporting that can be addressed in the future.
Conclusion
RDD is a valuable tool in biomedical research that allows scientists to make informed decisions about treatments, interventions, and policies. By comparing outcomes on either side of a threshold, researchers gain insights into the effectiveness of various healthcare strategies.
Choosing the right study design is critical for obtaining high-quality evidence. Get help from an expert biostatistician under Editage’s Statistical Analysis & Review Services.
Comments
You're looking to give wings to your academic career and publication journey. We like that!
Why don't we give you complete access! Create a free account and get unlimited access to all resources & a vibrant researcher community.
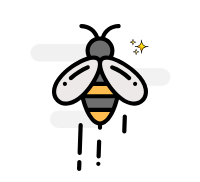
Subscribe to Conducting Research