What is propensity score weighting? A quick guide for biomedical researchers
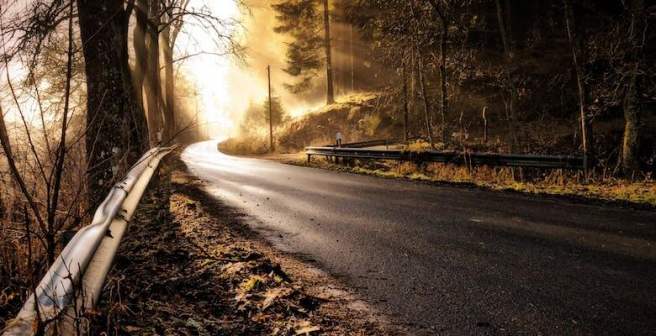
In observational studies where treatment assignment isn't random, it’s often challenging to compare groups and determine true differences between them. Enter propensity score weighting, a method that helps balance covariates between groups to draw more reliable conclusions. This blogpost will explain the principle behind propensity score weighting along with its advantages and disadvantages.
What is a Propensity Score?
The propensity score is the likelihood of receiving a treatment based on pre-treatment characteristics. It summarizes multiple variables into a single score, making group comparisons more straightforward.
Calculating Propensity Scores in Biomedical Research
Propensity scores are calculated using statistical models that predict the likelihood of receiving a treatment based on observed covariates. The process involves several steps:
- Selecting Covariates: Identify relevant variables that may influence treatment assignment. These are characteristics that differ between treated and untreated groups.
- Building a Logistic Regression Model: Use a logistic regression model to estimate the probability (propensity score) of receiving treatment based on the selected covariates.
- Obtaining Propensity Scores: After fitting the logistic regression model, each participant is assigned a propensity score, indicating their likelihood of receiving the treatment.
- Checking Balance: Evaluate whether the propensity scores effectively balance covariates between treated and untreated groups. Methods like standardized mean differences or histograms are commonly used for this assessment.
Propensity Score Weighting
Once we have the propensity scores, we assign weights to each observation based on how well it matches others in the opposite group. This ensures that groups are comparable, reducing biases.
Advantages of Propensity Score Weighting
- Balance Covariates: It helps create groups with similar characteristics, minimizing confounding variables.
- Reduced Selection Bias: By adjusting for confounding, propensity score weighting enhances the accuracy of treatment effect estimates.
- Flexibility: It can be applied to various study designs, making it versatile for different biomedical research scenarios.
Disadvantages of Propensity Score Weighting
- Assumption Reliance: Effective implementation relies on the assumption that all relevant covariates are measured and included.
- Modeling Challenges: Developing a robust propensity score model may be challenging, especially with large datasets or complex interactions.
- Sensitivity to Outliers: Extreme values in covariates can influence propensity score weighting results, requiring careful data examination.
Conclusion
Propensity score weighting is a valuable tool in biomedical data analysis, offering a practical approach to address confounding variables and improve the validity of observational studies. While it comes with assumptions and challenges, understanding its application can enhance the quality of research outcomes.
Boost the quality of data from your observational study, with the help of an experienced biostatistician. Check out Editage’s Statistical Analysis & Review Services.
Comments
You're looking to give wings to your academic career and publication journey. We like that!
Why don't we give you complete access! Create a free account and get unlimited access to all resources & a vibrant researcher community.
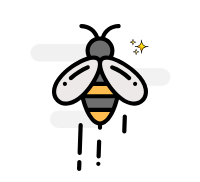
Subscribe to Conducting Research