Using bayesian statistics for meta-analysis: A handy guide for biomedical researchers
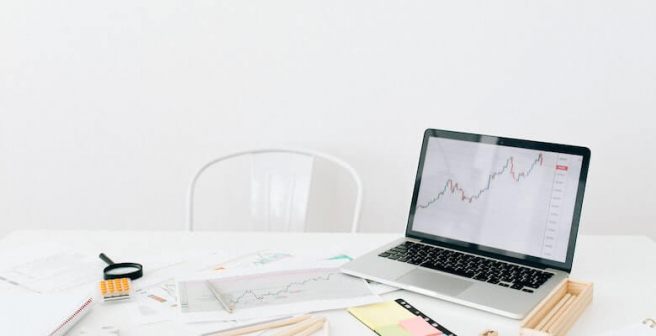
In biomedical research, making informed decisions is paramount. Meta-analysis---synthesizing data from multiple studies---is considered the gold standard for evidence-based decision making. But what if we told you there's a way to boost the value of the meta-analyses you conduct? Enter Bayesian statistics – a dynamic approach that goes beyond traditional methods. In this blogpost, we'll demystify how Bayesian statistics can revolutionize your approach to meta-analysis.
Prior Information
Bayesian meta-analysis starts with prior beliefs or information about the parameters being studied. In meta-analysis, this could be prior knowledge about the expected effect size or variability in the outcomes.
Likelihood Function
We then consider the likelihood of observing the data from each study given the parameters. This means we calculate how likely the study results are, assuming different values for the parameters.
Bayes' Theorem
Now, we use Bayes' theorem to update our prior beliefs based on the likelihood and the observed data. This gives us the posterior distribution, which represents our updated understanding of the parameters.
Pooling of Information
In meta-analysis, we have data from multiple studies. Bayesian statistics allows us to combine these studies by combining their likelihoods and updating our beliefs collectively. This pooling of information leads to more accurate estimates.
Uncertainty Quantification
Bayesian meta-analysis provides not just point estimates but also a range of possible values (credible intervals) for the parameters, which helps us understand the uncertainty in our conclusions.
Handling Heterogeneity
Biomedical studies can vary in terms of design, patient populations, and methods. Bayesian meta-analysis can model this heterogeneity, allowing for more realistic estimation of treatment effects while considering the differences between studies.
Model Flexibility
Bayesian methods allow for the incorporation of different types of information, like expert opinions or hierarchical structures in the data, making it adaptable to various meta-analytic scenarios.
Sensitivity Analysis
Bayesian meta-analysis also allows researchers to conduct sensitivity analyses, exploring how different prior assumptions impact the results, which can be valuable for assessing robustness.
Precautions in Bayesian Meta-Analysis
When you use Bayesian statistics for meta-analysis, it's essential to keep several precautions in mind to ensure the validity and reliability of the findings:
Prior Specification: Carefully select and justify your prior distributions. Improper priors can lead to biased results, so consider the impact of your prior assumptions on the outcome.
- Sensitivity Analysis: Perform sensitivity analyses by varying the prior specifications to assess the robustness of your results. This helps understand how different priors influence the conclusions.
- Publication Bias: Be aware of publication bias. Bayesian methods can help detect and correct for it, but it's crucial to assess the likelihood of unpublished studies or selective reporting.
- Convergence Checks: Ensure that Bayesian models have converged properly. Failure to do so may lead to unreliable estimates.
- Data Quality: Assess the quality and reliability of the data from individual studies. Low-quality data can introduce bias and negatively impact meta-analysis results.
- Transparency: Clearly document all aspects of your Bayesian meta-analysis, including prior choices, model specifications, and computational methods. Transparency enhances reproducibility and scrutiny.
Conclusion
By keeping these precautions in mind, you can leverage the power of Bayesian statistics for meta-analysis while maintaining the integrity of your findings. Meta-analysis is a valuable tool for healthcare professionals and researchers alike. By using Bayesian methods in meta-analysis, you can unlock new insights and make more-informed decisions for better healthcare.
Bayesian statistics can be complex. Seek advice from statisticians or Bayesian experts if you're not experienced in Bayesian meta-analysis. Consult Editage’s Statistical Analysis & Review Services.
Comments
You're looking to give wings to your academic career and publication journey. We like that!
Why don't we give you complete access! Create a free account and get unlimited access to all resources & a vibrant researcher community.
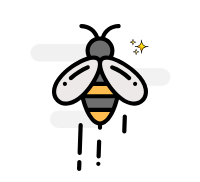
Subscribe to Conducting Research