Understanding Correlation Coefficients: A Comprehensive Guide for Researchers
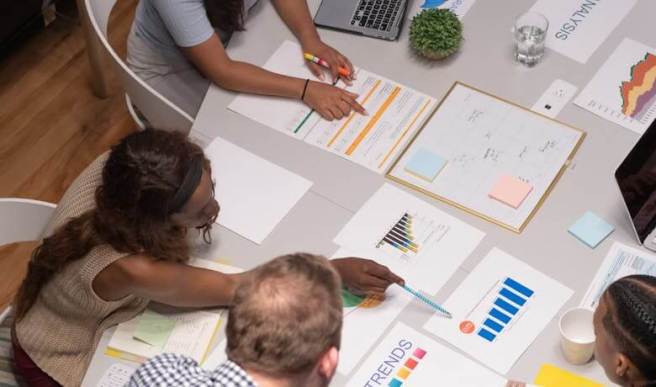
A common task in research is understanding how two variables are related to each other, such as amount of exposure to thirdhand smoke and asthma severity. When you’re analyzing data, correlation coefficients are valuable for quantifying the relationships between variables. In this blogpost, we’ll delve into these statistical measures and explore different types of correlations.
What are Correlation Coefficients?
Correlation coefficients quantify the strength and direction of the relationship between two variables. They provide insights into how changes in one variable may correspond to changes in another.
Types of Correlation: Positive vs. Negative
Positive Correlation: Indicates that as one variable increases, the other also tends to increase. For instance, LDL cholesterol levels and body mass index.
Negative Correlation: Suggests that as one variable increases, the other tends to decrease. An example would be insulin sensitivity and blood glucose levels.
Strength of a Correlation
Correlation coefficients are often categorized based on their strength:
Strong Correlation: Absolute values approaching 1 indicate a strong relationship between variables.
Moderate Correlation: Absolute values ranging from 0.5 to 0.7 suggest a moderate relationship.
Weak Correlation: Values closer to 0 imply a weak or negligible relationship between variables.
Pearson's r and Spearman's rho
Pearson's r: Widely used for assessing linear relationships between continuous variables. It ranges from -1 to 1, where 1 signifies a perfect positive linear relationship, -1 indicates a perfect negative linear relationship, and 0 suggests no linear relationship.
Spearman's rho: Suitable for assessing monotonic relationships, which may not necessarily be linear. It's robust to outliers and works well with ordinal or ranked data.
Precautions for Correlation Analysis
While correlation analysis offers valuable insights, it's crucial to approach it with caution:
Correlation Does Not Imply Causation: Establishing correlation does not confirm causation. Other factors may influence the observed relationship.
Sample Size Matters: Larger sample sizes enhance the reliability of correlation analysis, reducing the impact of outliers.
Consider the Context: Ensure variables are relevant to your research question. Including irrelevant variables can lead to spurious correlations.
Conclusion
Correlation coefficients are useful for researchers seeking to understand relationships between variables. By comprehending the nuances of positive and negative correlations, employing appropriate measures such as Pearson's r or Spearman's rho, and exercising caution in interpretation, researchers can harness the power of correlation analysis effectively in their studies.
Comments
You're looking to give wings to your academic career and publication journey. We like that!
Why don't we give you complete access! Create a free account and get unlimited access to all resources & a vibrant researcher community.
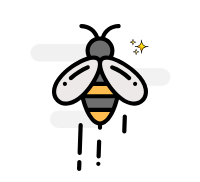
Subscribe to Conducting Research