The null hypothesis: What researchers often get wrong
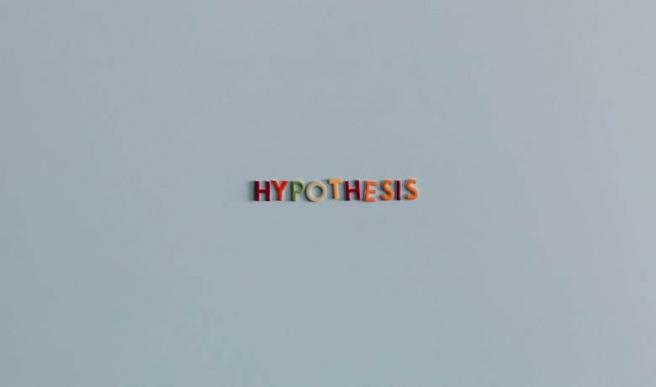
For biomedical researchers, it’s as important to understand basic statistical concepts as it is to understand cellular pathways. Among these concepts, one of the most important is that of a null hypothesis. Often denoted as H0, the null hypothesis is the default assumption in common statistical tests like ANOVA, Pearson’s correlation analysis, t-test, etc.
Null hypothesis: What does it mean?
The null hypothesis for a statistical test posits that there is no statistically significant difference, effect, or relationship between the variables under investigation. Basically, any observed differences, relationships, or effects are due to chance.
Alternative hypothesis: What we really want to know
The counter of the null hypothesis is the alternative hypothesis, often denoted as H1. The alternative hypothesis is usually the question we are interested in: that the relationship, difference, or effect observed in our data is not due to chance (i.e., it is genuine).
P values: Their pivotal role in testing the null hypothesis
A p-value quantifies the strength of evidence against the null hypothesis. It represents the probability of obtaining results as extreme as or more extreme than the observed data, assuming the null hypothesis is true. A lower p-value indicates stronger evidence against the null hypothesis, typically signifying statistical significance.
Note that a low p-value doesn’t mean that the alternative hypothesis is true. It simply means that that the results you’ve got are unlikely to have occurred merely due to random chance.
Null Hypothesis Significance Testing (NHST)
NHST is a method of statistical inference in which we decide whether the null hypothesis can be rejected or not.
Imagine you’re testing a new antihypertensive agent: the null hypothesis says it doesn't lower blood pressure. You collect data and calculate a p-value, which measures how likely your results are if the agent had no effect. A low p-value suggests your findings are unlikely to happen by chance, supporting the idea that the agent does lower blood pressure. NHST helps researchers make confident conclusions about their discoveries, ensuring that what they find is real and not just a fluke.
However, NHST has some widely documented shortcomings: Firstly, it focuses solely on statistical significance, ignoring effect size and practical relevance. A small p-value may indicate significance, but it doesn't necessarily mean the effect is meaningful in real life. Another drawback of NHST is that it dichotomizes results into significant or non-significant, leading to misinterpretation and publication bias, where statistically significant findings are more likely to considered important and to be published. NHST also relies on arbitrary thresholds like 0.05, which can be misused to support weak or spurious claims. Nevertheless, despite these shortcomings, NHST is still considered a pillar of statistical inference (Nulty, 2022).
Errors in hypothesis testing
During hypothesis testing, there’s a possibility of two kinds of errors:
Type 1 Error: False Positive
A Type 1 error occurs when we reject the null hypothesis when it is actually true. In simpler terms, it’s a false positive result. This error could lead researchers to believe there is a significant effect or relationship when, in reality, there isn’t.
Type 2 Error: False Negative
On the other hand, a Type 2 error occurs when we fail to reject the null hypothesis when it is false. In other words, we overlook a genuine effect or relationship, leading to a false negative result.
Researchers have to guard against both Type 1 and Type 2 errors. Type 1 errors can lead to spurious claims (e.g., that an intervention is effective when it actually isn’t) and Type 2 errors could result in missed opportunities for identifying crucial interventions or insights.
Summing up
If you heavily rely on conventional (frequentist) statistical tests like t-tests or ANOVAs in your research, it’s important to understand the principles behind the null hypothesis and NHST. Keeping in mind the shortcomings of NHST will help you guard against exaggerating or misinterpreting your results, and guide you in crafting a balanced and insightful report of your research.
Comments
You're looking to give wings to your academic career and publication journey. We like that!
Why don't we give you complete access! Create a free account and get unlimited access to all resources & a vibrant researcher community.
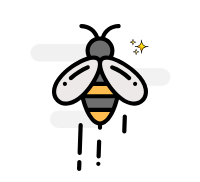
Subscribe to Conducting Research