Taming outliers in biomedical research: A handy guide
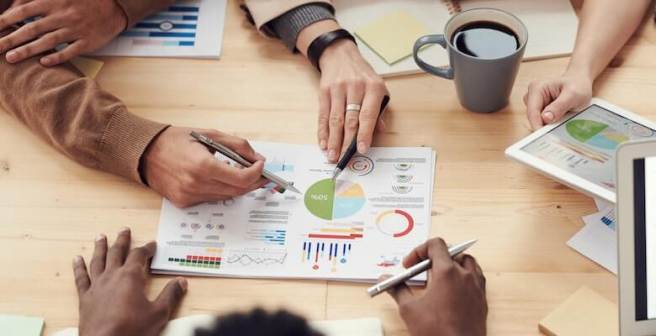
Outliers are those rebels in our data that refuse to conform. In the realm of biomedical research, where precision is key, these data points can be a source of both frustration and enlightenment. But what exactly are outliers, and how can we handle them effectively? Let’s embark on a journey through the world of outliers and discover the best practices in handling them.
What Are Outliers, Anyway?
Imagine you’re conducting a clinical trial to assess the effectiveness of a new drug. Most of your patients experience a moderate reduction in symptoms, but one patient shows an extraordinary improvement that’s far beyond the norm. That extraordinary improvement is an outlier, a data point that deviates substantially from the majority.
In biomedical research, outliers can manifest in various ways. For instance, they might be a subject with an unusually rapid response to treatment, an unexpected spike in a biomarker, or an outlier could be an error in data collection or entry.
Why Are Outliers Important?
Outliers are like signposts in your data. They can reveal hidden insights, anomalies, or even errors in your research. Let’s say you’re studying the effects of a new vaccine, and you notice an outlier in the group that received the vaccine. This outlier might indicate a unique response that warrants further investigation, potentially unveiling a new avenue of research.
Best Practices for Handling Outliers
Identify Them Carefully: Start by plotting your data visually. Box plots, scatter plots, or histograms are your friends. These visualizations can make outliers stand out, allowing you to identify them.
Example: In a study on blood pressure, if most subjects fall in the range of 110-130 mm Hg, a reading of 200 mm Hg is a glaring outlier.
Understand the Context: Before taking any action, consider the context of your study. Is the outlier a true observation, an error, or a sign of something extraordinary?
Example: In genetic research, an outlier might be a rare genetic mutation that causes a unique disease phenotype.
Choose the Right Approach: Depending on the situation, you can handle outliers in several ways:
Exclude Them: If an outlier is clearly erroneous (e.g., a data entry error), it’s acceptable to exclude it from your analysis.
Transform Data: In some cases, transforming your data (e.g., taking the logarithm) can reduce the impact of outliers.
Use Robust Methods: Consider using robust statistical methods that are less influenced by outliers. We’ll explore these further in later on in this blogpost.
Report Transparently: Whether you keep, exclude, or transform outliers, always report what you did and why. Transparency is essential in research.
Example: "One outlier with a systolic blood pressure of 200 mm Hg was excluded due to data entry error."
Statistical Tests That Can Handle Outliers
Let’s look at some statistical tests you can use when you’ve found outliers in the data and it’s not possible to exclude or transform them.
Mann-Whitney U Test: This non-parametric test is a robust alternative to the t-test for comparing two independent groups. It works well when you suspect non-normality or have outliers in your data. Instead of relying on means and variances, it compares the ranks of observations, making it resistant to extreme values.
Kruskal-Wallis Test: The Kruskal-Wallis test is an extension of the Mann-Whitney U test and is used to compare three or more independent groups. It assesses whether the medians of the groups are significantly different without assuming normality or equal variances, making it robust to outliers.
Spearman’s Rank Correlation: Spearman’s rank correlation coefficient is a non-parametric measure of the strength and direction of a relationship between two variables. It’s robust to outliers because it relies on the ranks of data points rather than their actual values.
Robust ANOVA: Robust ANOVA methods, such as the Brown-Forsythe or Welch ANOVA, are alternatives to traditional ANOVA that are less sensitive to outliers and heteroscedasticity. They can be used to compare means across multiple groups, even when there are outliers present.
Robust Regression: Robust regression techniques are designed to minimize the impact of outliers on regression models. One common method is the Huber loss function. Unlike ordinary least squares (OLS) regression, which gives equal weight to all data points, robust regression assigns different weights to data points based on their influence. Outliers receive lower weights, reducing their impact on the model. This approach helps maintain the accuracy of the regression model even in the presence of outliers.
Quantile Regression: Quantile regression extends traditional linear regression by estimating multiple quantiles of the response variable instead of just the mean. This makes it robust to outliers because it focuses on different parts of the data distribution. When you encounter outliers, quantile regression can provide valuable insights into how the relationship between variables changes at different quantiles. It’s particularly useful when you suspect that outliers affect certain percentiles differently.
Bootstrap Resampling: Bootstrap resampling is a versatile method for estimating confidence intervals and conducting hypothesis testing. It resamples your data by creating multiple simulated datasets, allowing you to assess the robustness of your results to outliers. You can use bootstrapping in conjunction with various statistical tests and regression models to account for outliers.
Bayesian Methods with Robust Priors: Bayesian methods inherently provide a framework for handling outliers. By specifying robust prior distributions, you can account for the possibility of outliers in your data. Bayesian methods allow you to express uncertainty in your model parameters, which can be especially valuable when dealing with data that may contain outliers.
In Bayesian frameworks, you can also incorporate outlier-detection models as part of the analysis. This involves modeling the presence or absence of outliers explicitly, giving you more control over their influence on the final results.
Conclusion
Outliers are not the enemy; they are valuable pieces of information waiting to be understood. By following the best practices outlined in this blogpost, you can leverage the power of outliers to uncover new research questions and unexplored research avenues. Remember, outliers are not always something to fear; they might just be the key to unlocking new discoveries in your field.
Do you want expert advice on what to do with the outliers in your own dataset? Consult a biostatistician under Editage’s Statistical Analysis & Review Services.
Comments
You're looking to give wings to your academic career and publication journey. We like that!
Why don't we give you complete access! Create a free account and get unlimited access to all resources & a vibrant researcher community.
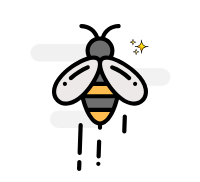
Subscribe to Conducting Research
Conducting research is the first and most exciting step in a researcher's journey. If you are currently in this stage of your publishing journey, subscribe & learn about best practices to sail through this stage and set yourself up for successful publication.