Statistical solutions to overcome missing data in clinical trials and observational studies
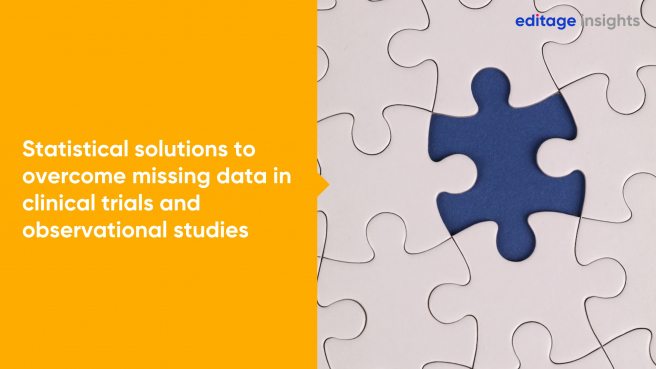
Data completeness is a critical aspect of observational studies and clinical trials, as missing or incomplete data can significantly impact the validity and reliability of study findings. Missing data can arise due to various reasons, such as participant dropouts, data collection errors, or incomplete responses. While approaches to handle missing data like last observation carried forward and mean substitution are popular owing to their ease, they are subject to numerous errors, resulting in unreliable data.i,ii It is essential for researchers to be well-versed in appropriate statistical methods that can effectively address missing and incomplete data to ensure the robustness of their study results. This blogpost introduces various statistical methods you can use to mitigate the challenges of missing and incomplete data in observational studies and clinical trials.
Multiple imputation
Multiple imputation is a statistical method that involves creating multiple plausible imputed datasets to account for missing data. It is based on the assumption that the data are missing at random (MAR), meaning that the probability of data being missing is dependent only on observed data and not on unobserved data. Multiple imputation can be performed using various techniques, such as regression imputation, Bayesian imputation, or predictive mean matching. The imputed datasets are then analyzed using standard statistical methods, and the results are combined using specialized rules to obtain final estimates and standard errors. Jakobsen et al. (2017) provide a practical guide to when and how multiple imputation can be used for missing data in clinical trials, including flowcharts.
Maximum likelihood estimation
Maximum likelihood estimation (MLE) is a statistical method that estimates model parameters based on the likelihood function of the observed data. MLE can be used to handle missing data by incorporating the likelihood of both observed and missing data in the estimation process. The Expectation-Maximization (EM) algorithm is a commonly used approach for implementing MLE in the presence of missing data. The EM algorithm iteratively estimates the missing data and updates the model parameters until convergence is achieved. Baker (2019) explains in detail how MLE can be used for partially missing outcomes.
Full information maximum likelihood
Full Information Maximum Likelihood (FIML) is another statistical method that can be used to address missing data. FIML estimates the model parameters by maximizing the likelihood function of the complete data, which includes both observed and missing data. Unlike traditional imputation methods, FIML does not require imputing missing data or creating multiple imputed datasets. Instead, FIML directly estimates the model parameters using all available data, including the incomplete data, which can result in more efficient and unbiased estimates. Li and Stuart (2019) discuss the use of both multiple imputation and FIML for missing data in randomized controlled trials.
Sensitivity analysis
Sensitivity analysis is a statistical method that assesses the robustness of study findings to potential changes in assumptions or imputation methods. It involves varying the imputation methods or assumptions of the missing data mechanism to evaluate the impact on study results. Sensitivity analysis can provide insights into the stability and generalizability of study findings and help researchers identify potential sources of bias or confounding due to missing data. Staudt et al. (2022) provides a detailed demonstration of how sensitivity analyses can be conducted for missing data in clinical trials.
Pattern mixture models
Pattern mixture models are statistical methods that account for different missing data patterns, such as missing completely at random (MCAR), missing at random (MAR), and missing not at random (MNAR). These models allow for different imputation methods or assumptions of the missing data mechanism depending on the missing data pattern. Pattern mixture models can be used to assess the impact of different missing data mechanisms on study results and provide more robust estimates. Iddrisu and Gumedze (2019) describe the application of a patent mixture model to handle missing data from a longitudinal trial.vii
Joint modeling
Joint modeling is a statistical method that simultaneously models the outcome of interest and the missing data mechanism. It allows for the estimation of both the model parameters and the missing data mechanism in a single model. Joint modeling can provide more accurate estimates by accounting for the relationship between the outcome and the missing data mechanism, and it can also handle missing data in longitudinal or time-to-event data. Gabrio et al. (2021) explain the use of joint modeling for handling missing at random data in clinical trials.
Does your study have missing data? Do you want to determine the most appropriate method of handling missing data in your study? Get expert advice from our biostatisticians under Editage’s Statistical Analysis & Review Services.
Comments
You're looking to give wings to your academic career and publication journey. We like that!
Why don't we give you complete access! Create a free account and get unlimited access to all resources & a vibrant researcher community.
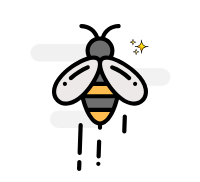
Subscribe to Conducting Research