Exploring the Intersection of AI and Knowledge Management in Academia: Insights from a Conversation with Mitja-Alexander Linss
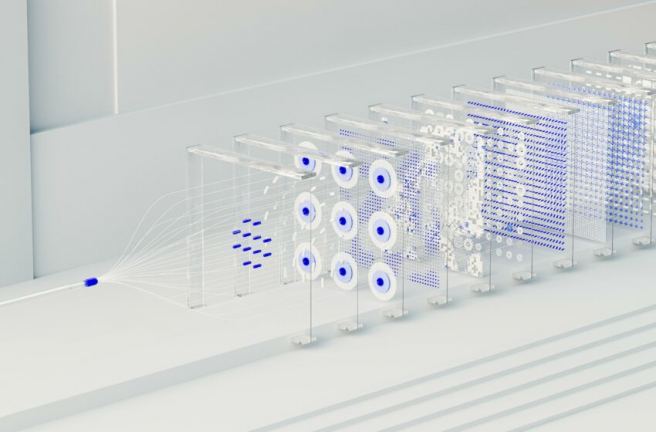
Editorial Note: The insights presented in this article are derived from the episode of the Insights Xchange podcast featuring Nikesh Gosalia, President, Global Academic and Publisher Relations at CACTUS, and Mitja-Alexander Linss, Chief Marketing Officer at Karger Publishers. This engaging discussion delves into the convergence of AI and knowledge management in academia, highlighting the inherent challenges and opportunities it presents. Furthermore, this conversation also explores the boundless potential that lies ahead with this powerful amalgamation.
With the ability to process and analyze huge amounts of data at unprecedented speeds, innovations and new fields in AI are transforming how different industries and organizations operate, making processes more efficient and improving decision-making at all levels. As AI continues to advance, it is more than likely to become even more ingrained in daily business operations.
The latest episode of the Insights Exchange podcast featured Mitja-Alexander Linss, Chief Marketing Officer at Karger Publishers. Mitja brings extensive experience in technology marketing and an acute grasp of the relationship between artificial intelligence and knowledge management in academia. In a discussion with Nikesh Gosalia, President of Global Academic & Publisher Relations at CACTUS, Mitja provides valuable insights into the transformation of knowledge management in the academic sector by AI. This engaging conversation explores the challenges and opportunities that AI presents in knowledge management processes.
How is AI transforming knowledge management?
Knowledge management is a crucial process for effectively organizing, capturing, and harnessing the collective knowledge of a group. The purpose is to ensure seamless storage and access to an organization's knowledge base, ultimately leading to enhanced efficiency and productivity. The rapid developments in AI bring about exciting possibilities for revolutionizing knowledge management systems for publishing and academic institutions.
Search and discovery: One area where this is particularly evident is in the realm of search and discovery. With the help of AI, traditional library platforms and conversational AI can be transformed into powerful tools for finding and accessing information.
Research integrity: The field of ethics compliance and plagiarism detection serves as a prominent area of emphasis for AI within the academic sector. With AI, we can effortlessly identify instances of plagiarism and fraudulent activity in academic submissions, safeguarding research integrity.
Accessibility: By leveraging natural language processing techniques, AI algorithms can analyze and summarize complex scientific articles. Furthermore, it can help in extracting relevant information effortlessly.
These advancements have the potential to revolutionize the way knowledge is shared and accessed, opening up new possibilities for research and learning. Mitja highlights several critical areas that deserve further exploration, “How is academic content is generated? How are research summaries and abstracts generated? And how can generative AI support the editorial process? And last but not least, data and analysis and visualization is a big area, of course. How to process and analyze vast amounts of research data, helping researchers identify trends and correlations and patterns. Other areas are general research support. How can we make research faster, more productive? How can we support researchers to be faster? How can the peer review process be faster and more effective?”
Tune in to the podcast episode now for more details.
Opportunities and Challenges in implementing AI for knowledge management in academia
With the wealth of data now easily accessible and the increasing computational power at our disposal, AI tools are gaining popularity in academia and publishing. Recognizing this, Mitja sheds light on some upcoming trends in AI systems for knowledge management in academia.
Evolving data models: Data models are undergoing a significant transformation, and these trends are set to have a monumental impact on the performance of AI in academic and publishing domains. Not only are we expecting data models to become even more massive, Mitja explains, “Perhaps 1,000 times larger than ChatGPT is today. But the more important development we're seeing is that small data models will evolve as they are cheaper and cover 99% of today's AI needs.” This shift highlights the growing importance of niche data models. Moreover, we are on the cusp of a new era with the emergence of multimodal models that seamlessly integrate text, figures, and images. This goes beyond generative AI on separate platforms, creating a truly enhanced AI experience. Additionally, the integration of these models with other tools, internet databases, and specialty software is poised to further enrich the landscape of AI.
Streamlining and enhancing peer review and editorial processes: One crucial area for advancement lies in leveraging AI tools to enhance academic editing and writing. Another aspect involves the potential for AI to handle peer review entirely, an intriguing question that has drawn attention. Other noteworthy opportunities are in finding ways to expedite the editorial process and reduce the need for manual checks in peer review while maintaining the quality of submissions.
Mitja adds, “Conversational search, generative and investigative AI, focusing on search and extraction are also becoming more important. AI-generated content, like lay and expert summaries, highlights are also something that we'll see more of.”
As AI applications in knowledge management evolve, the road ahead is not always straightforward. Embracing AI often demands substantial adjustments to established procedures, skills, and organizational frameworks. Prioritizing privacy and security while effectively managing the sensitivity of students' and research data is crucial. Mitja delves into the challenges that the scholarly publishing and academic ecosystem may encounter when integrating AI into their knowledge management systems. And just generally maybe privacy and security, sensitivity of data of students and research data that needs to be addressed.
Licensing of scientific data: Many large language models currently use scientific data for training without having clearly obtained copyright permissions beforehand. This raises numerous unanswered questions regarding how these models can comply with copyright requirements while using the scientific database as a foundation for training data. It is essential that the operators of large language models, such as OpenAI and others, actively engage in conversations with publishers to find resolutions for this matter. The question of copyright and the necessary permissions must be addressed and resolved in order to alleviate concerns surrounding this issue.
Bias and training data: AI algorithms can inadvertently adopt biases from their training data, which can lead to the unfair treatment of certain groups. While this issue is not new, it presents a significant challenge that must be addressed by academic institutions and all stakeholders in the publishing field. Although not a new problem, it presents a significant challenge that academic institutions and other stakeholders in the publishing industry must confront head-on. The solution lies in implementing proactive measures such as regular auditing. Another crucial step to mitigate bias would be to incorporate diverse training data and adhere to ethical guidelines to train AI models.
AI detection: The widespread usage of AI for AI detection has raised an important question: how can we distinguish scientific content created by AI? This issue gains even greater importance when we consider the potential for fraudulent use. For instance, incorporating AI into the peer review process holds immense promise, while on the other hand, it presents an opportunity for a surge in AI-facilitated fraud and plagiarism within the publishing field. It is becoming increasingly important for academic institutions to establish stringent guidelines to regulate the application of generative AI in research.
For more insights from Mitja, tune into the full conversation.
Recommendations for publishers and academic leaders looking to use AI to enhance knowledge management
There is a lot of enthusiasm and motivation when it comes to changing various processes within academia. As the industry forges forward to apply AI in their knowledge management systems, Mitja underscores, “It starts with understanding AI's potential and limitations. And develop a clear understanding of what AI can do and what it cannot do, equally important.” To help scholarly publishers and senior leaders in academia who are eager to improve knowledge management through the use of AI, Mitja offers some valuable recommendations on potential starting points and how to fully embrace and leverage this extraordinary opportunity.
- It is critical to establish clear objectives and priorities for AI implementation. A starting point would be to define specific goals to achieve. Ensuring that the initiatives align with the missions and needs of the organization’s mission is of absolute importance.
- Involving and engaging all available stakeholders should be a priority. Collaborating with faculty, researchers, librarians, and other key individuals will help in identifying the most urgent challenges and opportunities that can be addressed through the use of AI.
- Investing in AI expertise is a must, and this involves strategically developing or acquiring AI proficiency within one’s organization. Hiring skilled data scientists and AI engineers or collaborating with external experts is essential in order to harness the full potential of AI, enabling organizations to not only design but also effectively implement state-of-the-art AI solutions.
- High-quality data is essential for training AI models and making informed decisions. Prioritizing data quality and accessibility is necessary to ensure that the data used is clean, well-structured, and easily accessible in order to realize the full impact of AI.
- Ethical considerations play a crucial role in shaping such initiatives. It is imperative to develop clear guidelines and policies that align with the values of the institution or stakeholders involved. These should especially address concerns related to ethical conduct, data privacy, and potential biases.
- Lastly, but certainly not least, it is crucial to begin with modest undertakings and gradually expand as opposed to tackling projects that demand extensive resources and attention. It is highly advisable to initiate operations on a smaller scale and evaluate the impact before expanding.
Stream the full episode now to learn more.
Striking the right balance between human expertise and AI-driven knowledge management solutions
As AI becomes increasingly important, it is crucial for academic institutions and scholarly organizations to find innovative ways to use AI to enhance the learning experience, rather than to replace human expertise. As Mitja elaborates on his recommendations, he emphasizes, “AI is designed to support and assist humans, not replace them. So it's all about promoting a culture of collaboration between humans and AI systems and encourage the education of staff to view AI as a valuable tool that they can enhance their capabilities and productivity with.”
- Defining clear roles and responsibilities will clarify how AI can support, improve, or automate tasks, while humans continue to play crucial roles in decision-making and creativity.
- Ethical considerations and oversight are fundamental aspects that different stakeholders must take into account when it comes to the use of AI in academic settings. By establishing well-defined ethical guidelines for the use of AI in academic settings, we can ensure that decisions concerning AI implementation are in line with ethical standards, and that bias and discrimination are prevented. Oversight also plays a key role in this process, guaranteeing that a fair and unbiased approach is maintained throughout.
- It is essential to offer training resources to empower faculty and staff with AI literacy and proficiency. This includes fostering an understanding of the capabilities and limitations of AI tools, as well as teaching them how to effectively collaborate with AI-driven tools. The importance of continuous training cannot be overstated, as it will play an important role in consistently enhancing the capabilities of the academic workforce as the use of AI becomes more widespread.
- Transparency and explainability are also essential factors when it comes to AI-driven decision-making and recommendations. It is of utmost importance to ensure that the reasoning behind these AI systems is always transparent and can be readily explained. This promotes a sense of understanding and trust among people, which in turn enhances the effectiveness of collaboration.
Don’t miss the full podcast episode—listen now to dive into the topic.
It is clear that the convergence of AI and knowledge management in academia presents immense potential. With these technologies, organizations can completely transform the future of knowledge management, making it more dynamic and efficient.
Comments
You're looking to give wings to your academic career and publication journey. We like that!
Why don't we give you complete access! Create a free account and get unlimited access to all resources & a vibrant researcher community.
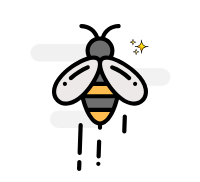