Ensuring parity between experimental and control groups: Useful statistical techniques researchers need to know
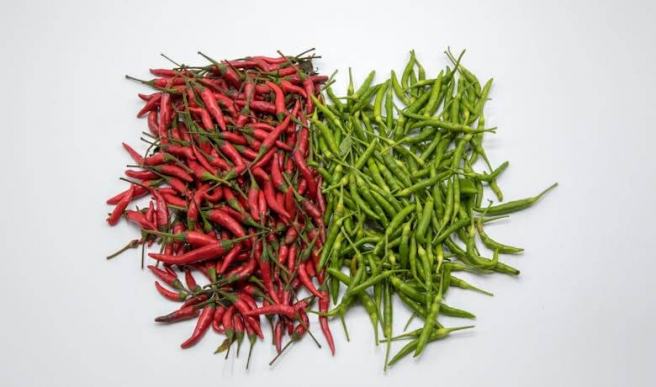
What are experimental and control groups?
In your study, you often need to divide your sample into two or more groups. You administer the intervention or “experiment” on one group, and compare their results with those of the other group (your “control” group). The group that receives the intervention or the variable you are testing is sometimes termed the “experimental group.”
This sounds pretty straightforward, right? But if the experimental and control groups are not similar to each other, you have some more variables (“confounders”) that can affect your results. For example, suppose you’re examining the effects of classical music on short-term memory. Your experimental group listens to Mozart and Beethoven before performing a task designed to test short-term memory performance. The control group doesn’t listen to any music before completing the same task. If the experimental group performs better on average than the control group, you’ve got evidence that listening to classical music can improve short-term memory. However, if the experimental group was significantly younger than the control group, there’s another variable---age---that might have affected the experimental group’s performance. Here, age is a confounding variable, because it affects or obscures your results.
For this reason, if you want to compile high-quality and powerful evidence in your research, you need to ensure that your experimental and control groups are “matched” on all important variables. That’s why, we’ve put together a handy list of statistical techniques that you can use for this kind of matching and thus generate robust, high-quality statistical results.
Strategies to Balance Your Experimental and Control Groups
Randomization
Here, you randomly assign subjects to experimental and control groups. This method minimizes pre-existing differences between groups by relying on chance.
Propensity Score Matching
Using this method, you calculate the probability that each subject will be assigned to the experimental group based on observed characteristics, and then match subjects with similar probabilities from each group. Chen et al. (2022) have compiled some useful guidelines on using propensity score matching.
Exact Matching
Just what it sounds like: you have to match subjects in the experimental group to subjects in the control group who have exactly the same characteristics. This is somewhat like finding an identical twin for every member of your experimental group, and can be pretty difficult to implement in real life.
Coarsened Exact Matching
Easier to implement that exact matching, this method involves grouping subjects into bins based on their characteristics and then matching subjects from each bin. Ripollone et al. (2020) compared the utility of coarsened exact matching versus other techniques like propensity score matching and Mahalanobis distance matching, and found that although coarsened exact matching is not optimal, it is a useful alternative when there are less than 10 strong confounders in the study.
Mahalanobis Distance Matching
Mahalanobis distance measures similarity considering correlations among characteristics. When you’re using Mahalanobis distance matching, you have to use the Mahalanobis distance to find matches, and thus you’re taking into account the correlations between characteristics. Simply put, you match people by considering how certain traits relate to each other, not just how similar each trait is.
Inverse Probability Weighting
Inverse probability weighting ensures groups are comparable by assigning weights to each subject based on their likelihood of being in the experimental or control group. This way, subjects less likely to be in their group are given more weight, balancing the groups and making comparisons fairer and more accurate. If you’re interested in getting started with this method, you can refer to Chesnaye et al. (2021), especially for longitudinal or observational research.
Comments
You're looking to give wings to your academic career and publication journey. We like that!
Why don't we give you complete access! Create a free account and get unlimited access to all resources & a vibrant researcher community.
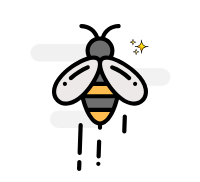
Subscribe to Conducting Research