Enhancing Healthcare: Power of Bayesian Methods in Personalized Medicine
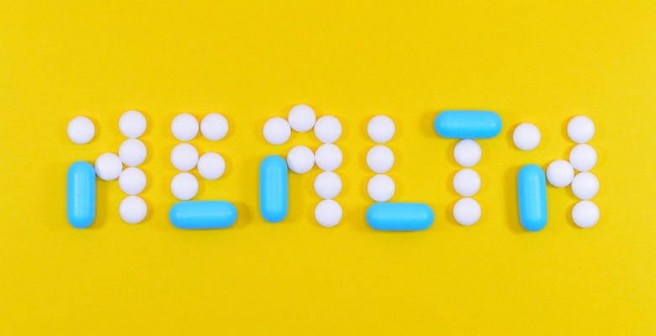
In healthcare, the quest for personalized treatment strategies tailored to individual patients has been a longstanding goal. With advancements in technology and data analytics, personalized medicine has witnessed a surge in interest, offering the potential to revolutionize patient care. Among the various methodologies driving this revolution, Bayesian methods stand out for their ability to integrate prior knowledge, accommodate uncertainty, and provide robust inference tailored to individual patient characteristics.
Understanding Personalized Medicine
Personalized medicine aims to move away from the one-size-fits-all approach to treatment, recognizing that individuals respond differently to medications and therapies due to genetic, environmental, and lifestyle factors. By leveraging insights from genetics, biomarkers, imaging, and other patient-specific data, personalized medicine seeks to optimize treatment outcomes, minimize adverse effects, and enhance overall patient well-being.
The Bayesian Paradigm: From Theory to Practice
At the heart of Bayesian statistics lies the concept of conditional probability, where beliefs about uncertain parameters are updated based on observed data. This iterative process, known as Bayesian inference, provides a principled framework for incorporating prior knowledge, data, and uncertainty into statistical models. In the context of personalized medicine, Bayesian methods offer several advantages:
- Integration of Prior Information: Bayesian analysis allows researchers to incorporate existing knowledge, such as prior clinical findings or expert opinions, into the analysis. By combining prior beliefs with observed data, Bayesian models provide a coherent way to update our understanding of individual patient characteristics and treatment responses.
- Accounting for Uncertainty: In healthcare, uncertainty is pervasive, whether it pertains to diagnostic accuracy, treatment efficacy, or patient outcomes. Bayesian methods offer a natural way to quantify and propagate uncertainty through the analysis, providing clinicians with more informative and nuanced insights into the risks and benefits associated with different treatment options.
- Individualized Risk Assessment: Bayesian models facilitate the estimation of patient-specific risks and probabilities, enabling clinicians to tailor treatment decisions to the unique characteristics and preferences of each patient. Whether predicting the likelihood of disease progression, treatment response, or survival, Bayesian methods provide a personalized approach to risk assessment and decision-making.
- Dynamic Learning and Adaptation: Personalized medicine is an evolving field, with new data and insights continually reshaping our understanding of disease mechanisms and treatment strategies. Bayesian methods excel in this dynamic environment, allowing for seamless integration of new evidence into existing models through techniques such as Bayesian updating and sequential analysis.
Applications in Practice
The application of Bayesian methods in personalized medicine spans a wide range of domains, including:
- Pharmacogenomics: Predicting drug responses based on genetic variations and individual patient characteristics. See, for instance, the Bayesian estimation framework developed by Öztaner et al. (2015) or the multivariate Bayesian structured variable selection model proposed by Zhao et al. (2023).
- Risk Prediction: Estimating personalized risk scores for diseases such as cancer, cardiovascular diseases, and diabetes. One such example is Gupta et al. (2021)’s machine-learned Bayesian network model for cancer patients.
- Treatment Optimization: Identifying optimal treatment regimens tailored to individual patient profiles, considering factors such as drug interactions, comorbidities, and patient preferences. For instance, Takahashi and Suzuki (2021) created a Bayesian optimization design for identifying a single maximum tolerated dose combination.
- Clinical Trial Design: Designing adaptive clinical trials that dynamically adjust treatment allocations based on accumulating data, maximizing efficiency and ethical considerations. Hong et al. (2022) virtually re-executed a breast cancer trial with Bayesian adaptive designs, and found that such designs substantially improved statistical power or lowered the average sample size required.
Conclusion
As the era of personalized medicine continues to unfold, Bayesian methods offer a powerful toolkit for transforming healthcare delivery from a population-based approach to one that is truly patient-centered. By harnessing the principles of Bayesian inference, clinicians and researchers can unlock new insights into disease mechanisms, treatment responses, and patient outcomes, ultimately leading to more effective, efficient, and compassionate care.
In the quest for precision medicine, Bayesian methods serve as invaluable allies, guiding us towards a future where healthcare decisions are not only evidence-based but also tailored to the unique needs and characteristics of each individual patient.
Ready to leverage Bayesian methods in your next research project? Collaborate with an expert biostatistician under Editage’s Statistical Analysis & Review Services.
Comments
You're looking to give wings to your academic career and publication journey. We like that!
Why don't we give you complete access! Create a free account and get unlimited access to all resources & a vibrant researcher community.
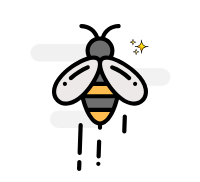
Subscribe to Conducting Research