Choosing between Frequentist and Bayesian statistics: a guide for biomedical researchers
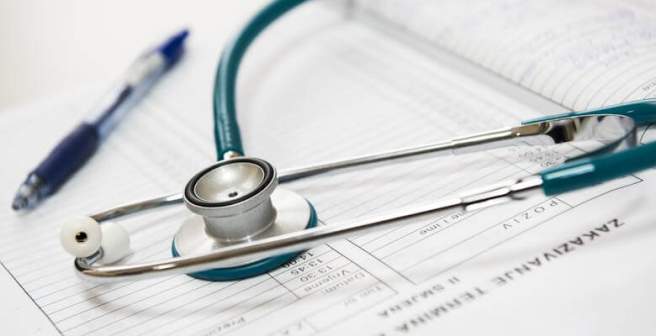
In the vast ocean of statistical methods, biomedical researchers often find themselves at a crossroads when choosing between traditional frequentist statistics and the increasingly popular Bayesian statistics. Let's embark on a journey to help you navigate these statistical seas and choose the right approach for your research questions.
Understanding the Basics
Frequentist Statistics
Frequentist statistics, the old stalwart, relies on probability and the concept of p-values. It's like a lighthouse guiding ships with the beacon of significance – a p-value less than 0.05 is often considered the safe harbor. Frequentist methods are deterministic, treating parameters as fixed and unknown.
Bayesian Statistics
On the other hand, Bayesian statistics takes a different tack. It embraces uncertainty by treating parameters as probability distributions. Bayesians use prior knowledge and update it with observed data to obtain a posterior distribution. No rigid cutoffs here – we get a range of possible parameter values, not just a single point estimate. Bayesian methods can also be used when existing knowledge is crucial; for example, using knowledge from clinical trials conducted among adults in order to infer safe and efficacious treatments for children, owing to the challenges associated with recruiting children for randomized controlled trials.
When to Choose Frequentist Statistics
1. Familiar Terrain
If you're comfortable with traditional methods and your audience is well-versed with hypothesis testing and p-values, frequentist statistics might be your anchor. It's the language many scientists have spoken for decades, making results more accessible to a larger number of researchers.
2. Simple Questions, Simple Answers
For straightforward research questions with clear hypotheses and well-defined populations, frequentist methods are often sufficient. For example, you could be comparing antibiotic use between pregnant women with and without gestational diabetes. Frequentist statistics are like a straightforward map for a straightforward journey.
3. Large Sample Sizes
Frequentist methods shine when you have large sample sizes. The Central Limit Theorem becomes your ally, and p-values tend to stabilize, giving you more confidence in your results.
Why Choose Bayesian Statistics?
1. Embracing Uncertainty
Bayesian statistics excels when dealing with uncertainty. If you have prior knowledge, or if your questions involve complex relationships, Bayesian methods allow you to incorporate this knowledge seamlessly. For instance, if you’re trying to predict cancer survival, as Liu et al. (2023) did, Bayesian methods allow you to explore various complicated relationships among different factors.
2. Small Sample Sizes
In the world of Bayesian analysis, small sample sizes are not as treacherous. Bayesian methods can provide more stable estimates by incorporating prior information, offering a lifeline when data is scarce. For example, if you’re studying a rare disease, the number of patients available might be limited.
3. Iterative Learning
If your research is an iterative process, with the need to continually update and refine your models as new data emerges, Bayesian methods provide a flexible framework. It's like having a ship that can adapt to changing winds. A clear example is the use of Bayesian adaptive designs for clinical trials, which allow researchers to save time and money while updating their knowledge about the success of the studied treatment.
Decision Time: Choosing Between Frequentist and Bayesian Methods
1. Evaluate Your Comfort Level
Consider your familiarity and comfort with the statistical methods. If you've been sailing with frequentist statistics for years and it serves your purpose, there's no need to jump ship.
2. Nature of the Research Question
Examine the nature of your research question. Is it straightforward or complex? Does it involve prior knowledge or is it a brave exploration into uncharted waters? Tailor your choice accordingly.
Conclusion: Collaborate and Seek Guidance
Both frequentist and Bayesian approaches offer unique advantages. The key is to navigate with purpose, choosing the methodology that aligns with your research goals, questions, and the nature of your data.
Don't embark on this journey alone. Collaborate with biostatisticians or researchers experienced in your chosen methodology. Seek guidance to ensure you're on the right course.
Get expert advice about whether you should use frequentist or Bayesian statistics in your next research project. Talk to a biostatistician under Editage’s Statistical Analysis & Review Services
Comments
You're looking to give wings to your academic career and publication journey. We like that!
Why don't we give you complete access! Create a free account and get unlimited access to all resources & a vibrant researcher community.
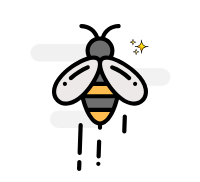
Subscribe to Conducting Research