Bootstrapping in biomedical research: A simple guide
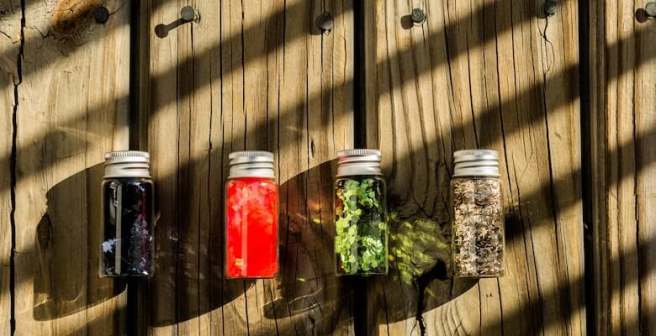
Biomedical research can be a complex puzzle, and sometimes, finding the right answer requires a bit of statistical magic. Enter bootstrapping! Bootstrapping is like making a lot of mini-teams from one big team. Imagine you have a bucket of different colored candies. You want to know the average candy color, but you can only pick a handful at a time. Bootstrapping helps you estimate that average by repeatedly picking candies and calculating the average over and over again.
Applying Bootstrapping in Biomedical Research
Let’s dive into a common scenario in biomedical research: omics data. Say you’re studying genes, and you’ve collected data from a group of patients. But you can’t study all patients due to time or resources. You might wonder, "Is my group representative of the whole population?"
Here’s where bootstrapping comes in. Instead of using the data from all patients, you create mini-samples from your group. These mini-samples simulate what might happen if you had data from everyone. You do this many times (hundreds or even thousands) to get a bunch of averages.
Now, what can you do with these averages? Well, you can calculate something called a confidence interval. It’s like saying, "I’m pretty sure the real average expression level of this gene falls between X and Y." The wider the interval, the less certain you are. Bootstrapping helps you figure out how much to trust your results.
Types of Bootstrapping Methods
There are a lot of different methods you can use for bootstrapping; here are the most commonly used ones:
Non-parametric Bootstrapping: This method repeatedly samples data from your actual dataset to create new "fake" datasets, helping you estimate the variability in your results without making assumptions about the data’s underlying distribution.
Parametric Bootstrapping: It assumes your data follows a specific statistical distribution (like normal or exponential) and generates many new datasets by tweaking the parameters of this assumed distribution. It’s useful when you have some knowledge about the data’s shape.
Bootstrapping Regression Models: This technique is used to assess the uncertainty in regression analysis, where you create new datasets by randomly selecting data points with replacement and rerunning your regression to understand how your results might vary.
Time Series Bootstrapping: In studies involving time-dependent data, this method accounts for temporal correlations by resampling entire time series chunks, maintaining the data’s sequential nature.
Bootstrap Hypothesis Testing: Rather than relying on traditional statistical tests, this approach generates a distribution of test statistics by resampling, allowing you to assess the likelihood of your results occurring by chance.
Stratified Bootstrapping: If your dataset has subgroups with different characteristics, stratified bootstrapping ensures that each subgroup is adequately represented in the resampled datasets, preserving the group-specific information.
Bayesian Bootstrapping: It combines bootstrapping with Bayesian statistics, enabling you to estimate uncertainty in a Bayesian context. It’s particularly useful when dealing with complex models and priors.
Why Bootstrapping is Handy
Sample Size Flexibility: Bootstrapping doesn’t care if you have a small dataset. It makes the most out of what you have.
Non-parametric Analysis: It’s great when your data doesn’t follow a normal distribution (common in omics studies).
Robustness: It can handle outliers or weird data points without losing its cool.
Visualizing Uncertainty: Bootstrapping provides a neat way to visualize your results, like error bars on a graph.
Disadvantages of Bootstrapping
While bootstrapping is a valuable statistical tool, it’s important to be aware of its limitations:
Computationally Intensive: Bootstrapping involves repeatedly resampling your data, which can be computationally demanding, especially with large datasets. It might not be suitable for all computing environments.
Assumes Independence: Bootstrapping assumes that your data points are independent of each other. In some cases, this assumption might not hold, leading to inaccurate results.
Bias: Bootstrapping can worsen bias if your original dataset is biased. It won’t magically correct for issues present in your initial data collection.
Precautions When Using Bootstrapping
To make the most of bootstrapping and avoid potential pitfalls, consider these precautions:
Address Bias: Deal with any bias in your data before applying bootstrapping. Bias can have a significant impact on the results.
Set the Right Resampling Size: Choose an appropriate resampling size (the number of iterations). While more iterations provide more reliable estimates, there’s a trade-off with computational cost.
Choose Your Bootstrapping Method Carefully: There are different types of bootstrapping (e.g., time series, stratified, or Bayesian bootstraps). Choose the one that best suits your data and research question.
Combine with Other Methods: Bootstrapping is often more powerful when combined with other statistical techniques. Don’t rely solely on bootstrapping; use it as a part of a broader analysis strategy.
Interpret Results Carefully: Be cautious when interpreting the results. Confidence intervals generated through bootstrapping can be wider than expected, so avoid overconfidence in your findings.
Remember that bootstrapping is a powerful tool, but like any statistical method, it should be applied thoughtfully and in the context of your specific research question and dataset. It’s not a one-size-fits-all solution, and understanding its limitations is crucial for using it effectively.
Ready to leverage the power of bootstrapping to make your analysis more reliable? Get guidance from an expert biostatistician under Editage’s Statistical Analysis & Review Services.
Comments
You're looking to give wings to your academic career and publication journey. We like that!
Why don't we give you complete access! Create a free account and get unlimited access to all resources & a vibrant researcher community.
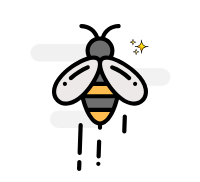
Subscribe to Conducting Research