Bayesian priors and prior distribution: Making the most of your existing knowledge
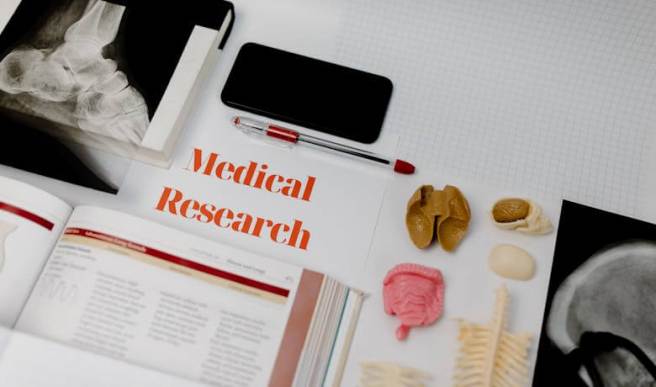
Bayesian statistics is increasingly popular in the biomedical sciences owing to its flexibility and ability to handle complex, high-dimensional data in addition to small sample sizes and/or missing/incomplete data. But when researchers start to dip their toes in Bayesian methods, they’re confronted by a very pressing question: What exactly are priors? And how do I choose a prior distribution?
Bayesian priors: The basics
“Priors” in Bayesian statistics refer to what you already know about the topic or question you’re investigating. Priors represent your initial beliefs or assumptions about the parameters of the model before observing any data. They encapsulate what we know about the parameters based on previous knowledge or expert opinion. Priors are typically specified using probability distributions.
Types of priors in Bayesian statistics
Informative Priors
An informative prior expresses specific, definite information about a variable. If prior information is available, you would choose a distribution that reflects this information. This could be based on previous studies, expert opinions, or theoretical considerations.
When you’ve solid, strong evidence to support your assumption (e.g., results of peer-reviewed studies), you use a strong prior. When you have limited information about a variable (e.g., subjective expert opinions without supporting data), you use a weakly informative prior.
Non-informative Priors
Non-informative priors are used if no prior knowledge is available or when aiming for objectivity. A non-informative prior is like saying, “I don’t know anything special about this situation, so let’s keep things as fair as possible.” It’s a way of being neutral and not favoring any particular outcome before seeing any data.
Regularizing priors
Also known as regularization priors, these are used to impose constraints or penalties on the parameters of a model. The purpose of a regularizing prior is to prevent overfitting and improve the generalization performance of the model by discouraging overly complex or extreme parameter values. In other words, regularizing priors keep a mathematical model from getting too complicated or extreme when you’re trying to predict things from data.
Regularizing priors are particularly useful when you’ve got models that have a large number of parameters relative to the amount of available data, because they help prevent the model from fitting the noise in the data too closely.
Because regularizing priors can play an important role in our Bayesian analysis, we’ll now take a look at the different types of regularizing priors.
- Laplace Prior: Also known as the double-exponential prior, the Laplace prior penalizes large parameter values by assigning higher probability density to values closer to zero. You’re basically telling the model “Keep the line as simple as possible, with not too many ups and downs.”
- Gaussian Prior: The Gaussian (normal) prior penalizes parameter values that deviate from a specified mean, effectively shrinking parameter estimates towards the mean. It’s like saying, “Try to keep the line close to the mean, don’t let it wander too far away.”
- Lasso Prior: The Lasso (least absolute shrinkage and selection operator) prior is a combination of the Laplace and Gaussian priors. It penalizes the absolute values of the parameters, promoting sparsity in the parameter estimates and encouraging some parameters to be exactly zero. It’s like saying, “Only use the important points to draw the line, ignore the less important ones.”
- Ridge Prior: The Ridge prior penalizes the squared values of the parameters, effectively shrinking parameter estimates towards zero while still allowing all parameters to be non-zero. You’re essentially telling the model “Don’t let the line get too tall or zigzag steeply, keep it as flat as possible.”
Prior distribution
This is the probability distribution representing our beliefs about the parameters before observing any data. Specifying priors in Bayesian statistics involves understanding the prior information available, selecting appropriate prior distributions, and considering their impact on the posterior distribution and final inference.
As mentioned earlier, Bayesian analysis allows for the incorporation of prior knowledge or information about the parameters. Selecting a prior distribution that accurately represents this prior knowledge can lead to more informative and meaningful posterior inferences.
The role of sensitivity analysis
When you’re using Bayesian statistics, it’s a good idea to conduct sensitivity analysis to evaluate how sensitive the posterior inference is to different prior specifications. This involves comparing results obtained with different priors to assess the robustness of the conclusions. If your results are sensitive to the choice of prior, you might need to rethink your approach. You could try gathering more data to reduce the influence of the prior, or you might need to choose a different prior that better reflects your beliefs or available information. Sensitivity analysis helps ensure that our conclusions are reliable and not just dependent on our initial assumptions.
Wrapping up
Carefully selecting a prior distribution helps maintain objectivity and transparency in your Bayesian analysis. Inappropriate or poorly chosen prior distributions can introduce biases into the analysis, leading to misleading conclusions. Therefore, it’s worth spending time in understanding how to choose priors and specify prior distributions, so that you can incorporate prior knowledge into your analyses in a meaningful and effective way.
Interested in getting started with Bayesian statistics? Consult an expert biostatistician, through Editage’s Statistical Analysis & Review Services
Comments
You're looking to give wings to your academic career and publication journey. We like that!
Why don't we give you complete access! Create a free account and get unlimited access to all resources & a vibrant researcher community.
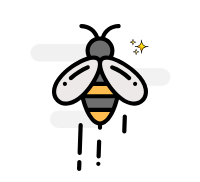
Subscribe to Conducting Research