An introduction to Principal Components Analysis for biomedical researchers
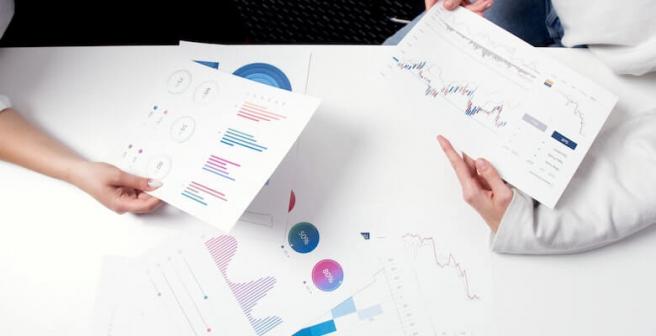
Handling large datasets, such as those in omics research, can be a daunting task. This is where Principal Components Analysis (PCA) comes to the rescue. PCA is a powerful tool that helps you make sense of big data, uncover hidden patterns, and reduce complexity. In this blogpost, we’ll break down PCA in simple terms, explore its applications in biomedical research, discuss its advantages and disadvantages, and share some crucial precautions to consider before diving into PCA.
Understanding Principal Components Analysis
PCA is a mathematical technique used in data analysis to simplify complex datasets by identifying and representing the most important patterns or dimensions. It reduces data dimensions while preserving critical information, making it easier to visualize and analyze large datasets, such as those in omics research.
Steps of Principal Components Analysis
Here are the essential steps involved in performing PCA:
Data Preprocessing: Standardize or normalize the data to ensure all variables have the same scale. This step is crucial as PCA is sensitive to the scale of the variables.
- Covariance Matrix Computation: Calculate the covariance matrix of the standardized data. This matrix represents the relationships between variables, showing how they co-vary.
- Eigenvalue and Eigenvector Calculation: Compute the eigenvalues and corresponding eigenvectors of the covariance matrix. Eigenvalues represent the variance explained by each principal component (PC), and eigenvectors represent the direction of each PC.
- Sort Eigenvalues: Arrange the eigenvalues in descending order. This step helps you prioritize which principal components to retain since they explain the most variance in the data.
- Select Principal Components: Decide how many principal components to retain based on your desired level of data dimensionality reduction. Typically, you aim to retain a sufficient number to explain a high percentage (e.g., 95%) of the total variance.
- Create the Projection Matrix: Form a matrix by selecting the top eigenvectors corresponding to the retained eigenvalues. This matrix serves as a transformation matrix to project the original data into the reduced-dimensional space.
- Transform Data: Multiply the original data by the projection matrix to obtain the new dataset in the reduced-dimensional space.
- Interpret Results: Analyze the transformed data to understand the contribution of each retained principal component. Higher eigenvalues imply more information retention.
Applications of Principal Components Analysis in Biomedical Research
- Dimensionality Reduction
Biomedical researchers often deal with datasets that have a vast number of variables (genes, proteins, patient attributes). PCA can help you reduce this complexity by identifying the most critical variables that capture the essence of your data. It’s like simplifying a recipe by using only the essential ingredients.
- Data Visualization
Visualizing complex data is crucial for insights. PCA can transform your high-dimensional data into a lower-dimensional space while preserving the most critical information. This means you can create easy-to-understand graphs and plots, making it simpler to spot trends or differences between patient groups.
- Noise Reduction
Biomedical data can be noisy, filled with irrelevant information. PCA can clean up the noise by emphasizing the essential patterns and removing the distracting details. Think of it as filtering out static to hear a clear message on the radio.
- Image Analysis
In medical imaging, PCA can be applied to reduce the dimensionality of image data, making it more manageable for further processing or feature extraction.
- Multivariate Analysis
When studying complex interactions among variables in biology or medicine, PCA can simplify data to identify significant relationships or confounding factors.
- Data Preprocessing
PCA can be part of data preprocessing pipelines to standardize data, making it more suitable for subsequent analyses such as clustering or classification.
Advantages of Principal Components Analysis
Simplicity: PCA simplifies complex data, making it more manageable and interpretable.
Data Compression: It reduces the number of variables while preserving data integrity, saving computational resources.
- Visualization: It allows for easy data visualization, aiding in the identification of trends and outliers.
- Noise Reduction: PCA can filter out irrelevant noise, enhancing the quality of your analysis.
Disadvantages of Principal Components Analysis
Loss of Interpretability: Reduced dimensions can make it challenging to interpret the variables’ physical or biological meaning. PCA may also discard valuable information.
Assumption of Linearity: PCA assumes that data follows linear patterns, which might not always be the case in biomedical research.
Precautions before Running Principal Components Analysis
Before diving into PCA, consider these precautions:
Data Preparation: Ensure your data is clean, standardized, and missing values are addressed.
- Understand Your Data: PCA works best when you have a good grasp of your dataset’s characteristics.
- Normalization: Normalize your data to avoid the dominance of variables with larger scales.
- Choose the Right Components: Decide how many principal components to retain carefully. Balance between dimensionality reduction and information retention.
- Validation: Always validate your results with domain-specific knowledge and other statistical methods.
Conclusion
PCA is a valuable tool for biomedical researchers dealing with big data. It simplifies complexity, aids in data visualization, and reduces noise. However, it’s essential to be aware of its limitations and take precautions before applying PCA to ensure the accuracy and relevance of your findings. So, embrace PCA as your data’s guiding star, helping you navigate the vast universe of biomedical data.
Running principal components analysis for the first time? Get help from an expert biostatistician under Editage’s Statistical Analysis & Review Services.
Comments
You're looking to give wings to your academic career and publication journey. We like that!
Why don't we give you complete access! Create a free account and get unlimited access to all resources & a vibrant researcher community.
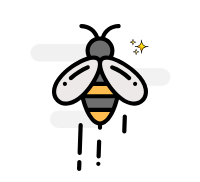
Subscribe to Conducting Research