An introduction to non-parametric tests for biomedical researchers
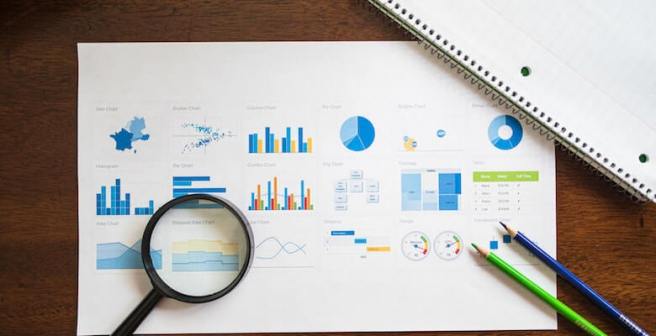
Statistical analysis is an integral part of biomedical research, but it’s often difficult to choose the right test to run. If you’ve ever encountered puzzling data distributions or outliers that just won’t behave, worry not! Non-parametric tests are your allies in such situations: they come to the rescue when your data isn’t playing by the rules of popular tests like t tests or ANOVAs. In this guide, we’ll delve into when and how to use these tests effectively, armed with examples from biomedical research.
Advantages and Limitations of Non-Parametric Tests
Non-parametric tests are flexible and robust, which makes them great for dealing with real-world, messy data. They don’t demand stringent assumptions, making them versatile tools in your statistical toolkit. However, they might be slightly less powerful than their parametric counterparts when your data is normally distributed and assumptions are met. Let’s look at the most popular non-parametric tests in biomedical research.
Mann-Whitney U Test: A Friend to Ordinal Data
Imagine you’re investigating levels of a specific clinical biomarker, NGAL, in people with and without with chronic kidney disease. When you collect the data, NGAL levels follow a skewed distribution. Here, the Mann-Whitney U test rides in on a white horse. It helps you compare these two groups without the need for a normal distribution, making it ideal for situations like these.
Wilcoxon Signed-Rank Test: A Pair-Wise Pal
Say you’re researching the impact of a dietary intervention on cholesterol levels. You have before-and-after measurements for each participant. Again, like in the earlier example, the data doesn’t follow a normal distribution, but the data are paired (i.e., related). No worries! The Wilcoxon Signed-Rank Test is designed for non-normally distributed paired data. It helps you determine whether the intervention led to a significant change, without the need for that pesky normality assumption.
Kruskal Wallis Test: Exploring Beyond Two Groups
What if you’re dealing with more than two groups? That’s where the Kruskal-Wallis test comes into play. Imagine you’re analyzing pain scores after three different treatments. If the scores aren’t normally distributed, the Kruskal-Wallis test steps up to help you figure out if there’s a significant difference among the treatments.
Time for Group Dynamics: Friedman’s ANOVA
Now, picture a study where you’re tracking blood pressure levels of patients across three time points. The data isn’t quite fitting the normal mold, but Friedman’s ANOVA is your solution. This test allows you to determine if there’s a significant difference in blood pressure over time without assuming a normal distribution.
Spearman’s Rho and Kendall’s Tau: Examining Relationships
Non-parametric tests also can be used to explore relationships between variables. Spearman’s rho is a good choice when you’re testing for correlations among ranked data. For instance, you could be assessing the correlation between ranked pain perception scores and genetic expression levels to explore genetic factors related to pain sensitivity.
On the other hand, Kendall’s tau helps you uncover whether the variables tend to move together in a consistent order, regardless of the specific values involved. It’s particularly handy when you have data where the order of values matters more than the specific numerical differences between them. Suppose you’re trying to understand patient preferences for different treatment methods for chronic pain. You ask patients to rank the effectiveness of three treatment options: medication, physical therapy, and acupuncture. By applying Kendall’s Tau, you can analyze the relationships between patients’ rankings of the treatments: do patients who rank one treatment method higher tend to rank the other treatments higher as well? Or do patients have distinct and opposite preferences for different treatment methods?
Conclusion
From wrangling ordinal data to handling outliers, non-parametric tests offer a robust alternative to traditional methods. Whether you’re diving into comparisons, exploring group dynamics, or teasing out relationships, non-parametric tests have your back. So, arm yourself with these tools and venture forth into your data analysis adventures with confidence!
Get expert advice on choosing and running the right statistical test for your data and research question. Check out Editage’s Statistical Analysis & Review Services.