An introduction to multiple imputation by chained equations for biomedical researchers
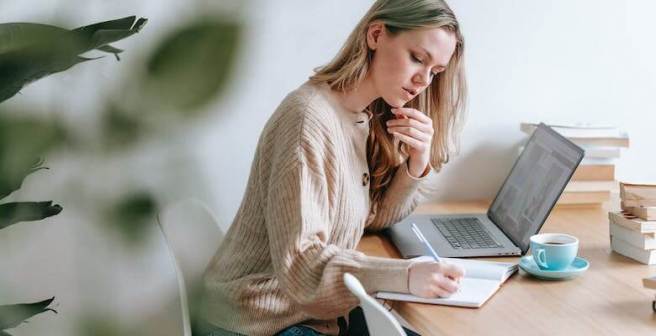
We all know the frustration of missing data—those elusive bits of information that decide to play hide and seek in our datasets. Biomedical research often deals with complex phenomena, and missing values can throw a wrench into our data analyses. Today, we're diving into the fascinating world of Multiple Imputation by Chained Equations (MICE)---which might just be the spell you need to tackle those pesky missing values. Let's break it down together.
What is MICE, Anyway?
Multiple Imputation by Chained Equations is not as complex as it sounds. It's a statistical method by which we can predict what's missing based on the information at hand. MICE imputes missing values by iteratively estimating them, taking into account the relationships between variables.
Breaking It Down: The Chained Equations
The "chained equations" part is where the magic happens. Instead of imputing all missing values at once, MICE takes a step-by-step approach. It starts by estimating one variable at a time, considering the observed data and the imputed values from the previous round. This iterative process repeats until the values settle into a harmonious dance, creating a complete dataset.
What happens during MICE?
If it’s your first time using MICE, you might find it a bit complicated. But follow the steps below, and you’ll soon use this technique with confidence:
Step 1: Identify Missing Data
Before diving into MICE, take a close look at your dataset and identify where the missing values lurk. Know your enemy, right?
Step 2: Understand Your Variables
Get to know your variables. MICE works best when it understands the relationships between them. Is there a connection between variable A and variable B? Knowing this helps MICE work its magic.
Step 3: Initialization
MICE starts by filling in missing values with some initial estimates. Think of this as the first draft of your data story. It might not be perfect, but it's a starting point.
Step 4: Iterative Imputation
Now, the magic begins. MICE doesn't tackle all missing values at once. It takes turns, starting with one variable and then moving on to the next. In each round, MICE estimates missing values based on observed data and imputed values from the previous round. It's like building a story chapter by chapter.
Step 5: Repeat Until Convergence
MICE repeats the imputation process until the values stop changing much—until your data story becomes stable. This ensures that the imputations have settled into a reliable pattern.
Step 6: Analyze and Pool
Once MICE has done its thing, you analyze each imputed dataset separately. Think of them as different versions of your story. Then, you pool the results to get one comprehensive tale. This pooling accounts for the uncertainty in the imputations.
Step 7: Assess the Results
Check the imputed datasets for consistency. Are they telling a coherent story? Assess the variability between them. MICE provides not just imputed values but also a measure of uncertainty, so you know how much faith to put in your results.
Step 8: Enjoy the Complete Dataset
Voilà! You now have a dataset with fewer gaps, and you're ready to embark on your analysis adventure with a more complete and robust set of data.
Why Choose MICE?
One word: flexibility. MICE is like the Swiss Army knife of imputation methods. It adapts to various types of missing data patterns, making it a versatile tool for different biomedical studies. Whether your missing data is missing completely at random or follows a more complex pattern, MICE is up for the challenge.
Getting Started: A Simple Example
Let's say you have a dataset with missing values in variables A, B, and C. MICE starts by estimating variable A, considering the observed data and imputing the missing values. Then, it moves on to B and C in subsequent rounds, creating a complete dataset that retains the complexity of your original data.
The Benefits of MICE in Biomedical Research
- Preserving Relationships: MICE doesn't just impute values blindly; it considers the relationships between variables, preserving the intricate web of connections in your data.
- Accurate Uncertainty Estimation: MICE doesn't just provide imputed values; it also gives you a measure of uncertainty. This is crucial in biomedical research, where understanding the reliability of your results is paramount.
- Enhancing Statistical Power: By salvaging incomplete data, MICE ensures you make the most of your dataset, boosting statistical power and potentially uncovering new insights in your findings.
Conclusion
So, there you have it—MICE, your new best friend in the battle against missing data. Next time those values decide to play hide and seek, arm yourself with MICE and watch the magic unfold in your dataset.
Struggling to cope with missing data? Our expert biostatisticians can help you with data cleaning and management. Check out Editage’s Statistical Analysis & Review Services.
Comments
You're looking to give wings to your academic career and publication journey. We like that!
Why don't we give you complete access! Create a free account and get unlimited access to all resources & a vibrant researcher community.
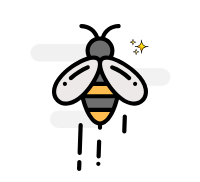
Subscribe to Conducting Research