All you need to know about repeated measures analysis in 5 minutes
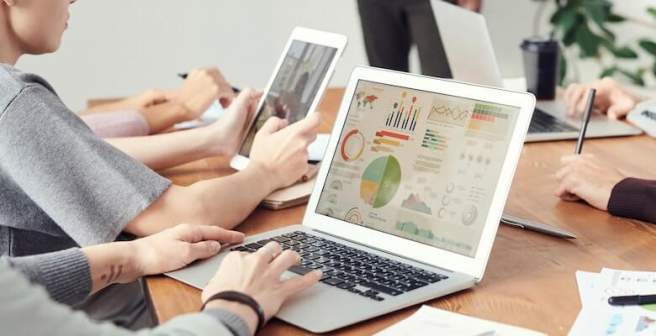
Have you ever wondered whether collecting data at multiple time points from the same set of subjects could introduce some bias into your results?
Well, repeated measures analysis is a statistical technique that allows us to examine changes in a variable over time while taking into account the correlated nature of the measurements. This method is especially useful when we have multiple observations from the same subjects, which is quite common in biomedical research.
Let’s say we have a cohort of individuals with diabetes, and we want to study the effect of a new medication on their blood glucose levels over time. We measure their blood glucose levels before starting the medication (baseline), and then at regular intervals (e.g., weekly) for a duration of three months.
Using repeated measures analysis, we can analyze the changes in blood glucose levels within each individual over time, while accounting for the correlation between the measurements. This analysis allows us to determine if the medication has a significant effect on reducing blood glucose levels compared to the baseline, considering the within-subject variability and any potential confounding factors. Instead of treating each measurement independently, repeated measures analysis considers the interdependency of the measurements, providing us with more accurate and powerful results. Some participants may be genetically predisposed to high blood glucose levels, while others may have more physically active lifestyles. In a repeated-measures analysis, we can see whether the medication changes blood glucose levels for these participants, considering what their previous levels were.
Benefits of Repeated Measures Analysis
Increased Precision: By accounting for the correlation between measurements, repeated measures analysis can improve the efficiency and precision of our statistical tests.
Reduced Variability: Accounting for within-subject variability can help us tease out the true changes over time, as it minimizes the impact of individual differences between participants. This is particularly valuable when we’re studying something that’s relatively subjective, such as pain perception or self-efficacy.
Enhanced Control: Repeated measures analysis allows us to control for confounding factors that remain constant within subjects, such as genetic predispositions or socioeconomic status. By doing so, we can gain a clearer understanding of the specific effects we’re interested in.
Making the Most of Repeated Measures Analysis
Here are some essential steps you must follow, to make the most of your repeated-measures analysis:
- Data Preparation: As with any statistical analysis, good data preparation is crucial. Make sure you have a well-structured dataset, with each row representing a unique subject and columns representing different time points or conditions. Also, you’ll need to take measures to deal with missing data, outliers, and potential sources of bias.
- Choosing the Correct Analysis: Repeated measures analysis offers various methods, depending on the nature of your data and research question. Some commonly used techniques include repeated measures ANOVA, linear mixed-effects models, and generalized estimating equations (GEE). Consult with a statistician to determine the most appropriate method for your study.
- Assumptions and Model Checking: Repeated measures analysis has certain assumptions that need to be met. These may include sphericity (equal variances between time points), linearity, and normality of residuals. Always check these assumptions and explore diagnostic plots to ensure the validity of your results.
- Interpretation: Once you’ve obtained your results, it’s time to interpret them in the context of your research question. Pay attention to effect sizes, confidence intervals, and p-values, but remember that statistical significance is not the only criterion for drawing conclusions. Consider the clinical or biological relevance of your findings and their implications for future research.
When You Should Avoid Repeated-Measures Analysis
Although repeated-measures analysis can be very useful, it’s not always the best solution to your statistical problems. You’ll need to exercise caution or avoid using repeated measures analysis in the following scenarios:
- Independence of Measurements: Repeated measures analysis assumes that measurements within the same subject are correlated. If the measurements are truly independent, a different analysis approach, like the independent samples t test, would be more appropriate.
- Violation of Assumptions: Repeated measures analysis has certain assumptions, including sphericity (equal variances between time points) and linearity. If these assumptions are violated, alternative methods, such as robust regression or non-parametric tests, may be more suitable.
- Incomplete or Uneven Data: Repeated measures analysis requires a complete set of measurements for each subject across all time points. If you have a lot of missing data or uneven measurement intervals, it may complicate the analysis or lead to biased results. In such cases, imputation techniques or alternative analytical methods should be considered.
- Different Sample Sizes: If the number of subjects or measurements differs substantially between time points or conditions, it can introduce biases in the analysis. So when your sample size varies a lot between measurements, specialized methods like weighted or mixed-effects models may be more appropriate.
- Violation of the Correlation Structure: Repeated measures analysis assumes a specific correlation structure, such as compound symmetry or autoregressive correlation (i.e., the variances of the differences between all possible pairs of measurements are equal, and the correlations between the measurements are the same). If the correlation pattern in the data deviates from these assumptions, it may impact the validity of the analysis. Exploratory analyses or alternative models that account for specific correlation structures may be more suitable.
Do you want to unlock the power of repeated-measures analysis and other valuable statistical techniques in your research? Consult an expert biostatistician under Editage’s Statistical Analysis & Review Services.
Comments
You're looking to give wings to your academic career and publication journey. We like that!
Why don't we give you complete access! Create a free account and get unlimited access to all resources & a vibrant researcher community.
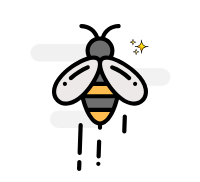
Subscribe to Conducting Research