A handy guide to Bayesian Neural Networks for biomedical researchers
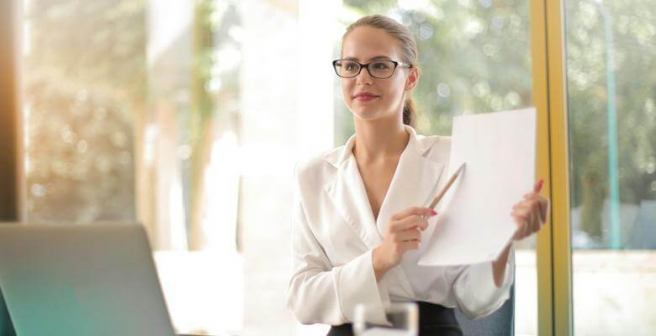
Neural networks are computational models inspired by the human brain’s structure and function. They consist of interconnected nodes, or neurons, organized into layers. These networks learn to perform tasks by adjusting the weights of connections based on input data. For example, neural networks analyze complex biomedical data, such as genetic information or medical images, to assist in disease diagnosis and prediction, drug discovery and development, image analysis, etc.
What are Bayesian Neural Networks?
Traditional neural networks provide deterministic outputs, giving a fixed prediction for a given input. In contrast, Bayesian neural networks introduce uncertainty into the mix. They use Bayesian statistics to model not just the most likely prediction but the entire distribution of possibilities. This is particularly useful in situations where data is scarce or noisy.
Advantages of Bayesian Neural Networks
Handling Uncertainty in Medical Predictions
In biomedical research, uncertainty is inherent. Patients vary, and experimental conditions fluctuate. Bayesian neural networks acknowledge this uncertainty, allowing researchers to better quantify and understand the limitations of predictions. This is crucial when dealing with intricate biological systems.
Enhancing Decision-making with Probabilities
Biomedical decisions often involve risk assessment. Bayesian neural networks provide a probabilistic framework, assigning likelihoods to different outcomes. This can aid researchers in making informed decisions, especially in areas like disease diagnosis or treatment planning.
Handling Limited Data
Biomedical datasets are often limited, making it challenging to train accurate models. Bayesian neural networks excel in such scenarios. By incorporating prior knowledge and expressing uncertainty, they mitigate the risk of overfitting to sparse data, making them valuable tools in the medical research toolkit.
Adaptability in Dynamic Environments
Biomedical systems are dynamic, subject to change over time. Bayesian neural networks can adapt to evolving data by continuously updating their beliefs. This adaptability is particularly advantageous when monitoring long-term health trends or studying dynamic biological processes.
Challenges and Considerations of Bayesian Neural Networks
While Bayesian neural networks offer significant advantages, they come with challenges. Training can be computationally intensive, and interpreting uncertainty requires careful consideration. Researchers must strike a balance between model complexity and practicality, considering the specific demands of their biomedical applications.
Conclusion
When you’re navigating the ever-evolving landscape of biomedical data, Bayesian neural networks are a promising ally. By embracing uncertainty and providing a nuanced perspective, these models contribute to more robust analyses and informed decision-making. As the field continues to progress, Bayesian neural networks hold great potential for advancing our understanding of complex biological systems.
Ready to leverage the power of Bayesian neural networks in your next research project? Take advice from an experienced biostatistician, under Editage’s Statistical Analysis & Review Services.