6 Common mistakes made by biomedical researchers while testing the relationships between variables
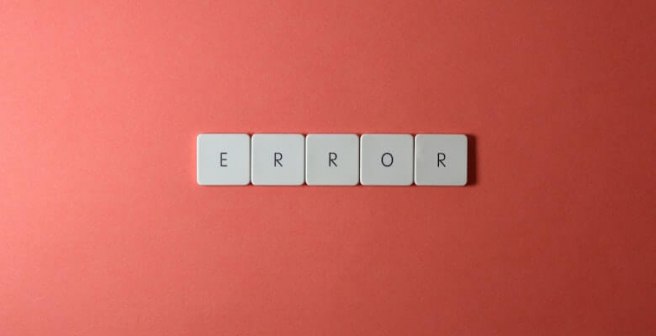
Typically, relationships between variables are tested using correlation, association, or regression analysis (we’ve covered special relationships like odds and risks in another post). Today, we’ll look at some important precautions for such analyses, to ensure your findings are reliable and provide high-quality evidence.
1. Not clearly identifying and summarizing the variables tested: Readers always need to know exactly what you’re studying and how you’ve summarized these variables. For example, if you’re examining the relationship between exercise and glucose control, you’ll need to define glucose control in the context of your study (are you considering HbA1c levels, post-prandial glucose levels, a combination of the two, etc.)?
2. Not checking data type: Before running any kind of analysis, you need to verify what type of data you’ve collected. Data can be continuous (e.g., BMI), categorical (e.g., race), or ordinal (e.g., cancer stage). The type of test you run depends on the variables you have. Correlations can be calculated between continuous variables, whereas tests of association (e.g., chi-square) can be calculated between categorical variables.
3. Not checking data distribution: Understanding distribution of diverse datasets can prevent misleading conclusions. By recognizing this, you can choose appropriate statistical tests or consider transforming the data to ensure accurate results. For example, Pearson’s r can be calculated if the two continuous variables are normally distributed, whereas Spearman’s rho may be a more suitable option for non-normally distributed variables.
4. Not checking for missing data and outliers: In any research study, missing data and outliers are sneaky little devils that can skew your analysis. Imagine you are investigating the relationship between age and cholesterol levels, using electronic health records (EHRs). You might find that some EHRs don’t include VLDL cholesterol levels, leading to missing data.
Addressing missing data and handling outliers with care is essential. You could use imputation methods to replace missing data or consider robust statistical techniques if outliers are present. Ignoring them or using inappropriate methods like Last Observation Carried Forward might introduce bias into your results, and we definitely don't want that!
5. Using vague descriptors: When it comes to presenting your findings, clarity is key! Using terms like "strong" or "weak" to describe relationships between variables can be vague and open to interpretation. Instead, report actual coefficients obtained from your analysis.
For example, if you are studying the correlation between exercise and cardiovascular health, mentioning a specific correlation coefficient (like Pearson's r) gives a clear picture of the relationship's strength and direction. This approach leaves no room for confusion and improves the rigor of your conclusions.
Pro tip: Don’t report just p values either! In both your abstract and main text, include correlation coefficients (with the – sign if needed), to give readers a clear picture of the key relationships you’re studying.
6. Drawing inferences beyond what the analysis may substantiate: Biomedical researchers use a variety of statistical methods, from regression analysis to t-tests and ANOVAs. Each analysis type yields specific insights, and it's essential to draw inferences accordingly.
Suppose you find that dietary fiber intake is inversely correlated with acne severity. Based on your findings, you could recommend that acne management programs include a component on getting participants to consume more fiber. However, you can’t claim that a low-fiber diet causes acne, because you’ve only done a correlation analysis.
Conclusion
So there you have it, six crucial precautions to keep in mind while testing relationships between variables in biomedical research. By following the above best practices, you'll be well-equipped to produce high-quality, robust research that advances our understanding of human health.
Our expert biostatisticians can help you make sure you're using the right methods to analyze relationships in your study, in keeping with your study design, research question, and data characteristics. Check out Editage's Statistical Analysis & Review Services.
Comments
You're looking to give wings to your academic career and publication journey. We like that!
Why don't we give you complete access! Create a free account and get unlimited access to all resources & a vibrant researcher community.
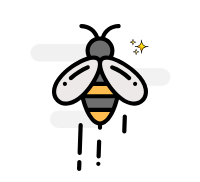
Subscribe to Conducting Research