5 Precautions Biomedical Researchers Should Take When Analyzing Data from a Large Public Registry
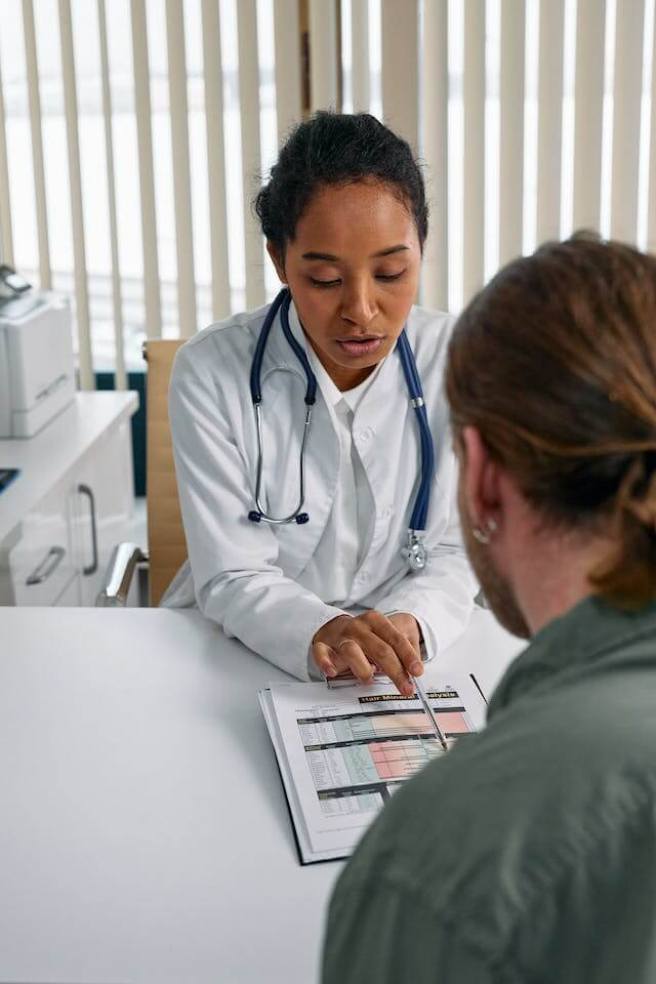
Biomedical researchers use large public registries for comprehensive and diverse datasets that facilitate population-scale studies. These repositories provide valuable information on diseases, treatments, and outcomes, enabling researchers to analyze trends, identify risk factors, and generate insights for evidence-based interventions, contributing to advancements in medical knowledge and public health. However, data from public registries is not without drawbacks, particularly bias, heterogeneity, missing data, inconsistencies, and privacy concerns. The robust interpretation of findings from large public registries requires a careful and systematic approach to statistical analysis. In this blogpost, we’ll discuss 5 important precautions biomedical researchers need to follow when analyzing data from a large public registry.
1. Data Quality and Reliability:
Biomedical researchers should be vigilant about the quality and reliability of the data obtained from a large public registry. Public registries may have diverse sources contributing data, and variations in data collection methods can introduce biases or errors. Researchers need to thoroughly assess the data quality, identify potential sources of bias or confounding, and implement appropriate measures to address or mitigate these issues.
2. Statistical Power and Sample Size:
Ensure that the sample size is adequate for the statistical analyses being performed. Large datasets do not guarantee statistical power if the sample size is not appropriately matched to the research questions. Biomedical researchers should conduct power analyses to determine the minimum sample size required to detect meaningful effects, minimizing the risk of drawing false conclusions due to insufficient statistical power.
3. Multiple Testing Corrections:
Large datasets often involve the analysis of numerous variables, increasing the likelihood of obtaining statistically significant results by chance (Type I errors). Biomedical researchers must apply appropriate corrections for multiple testing, such as Bonferroni or False Discovery Rate (FDR) corrections, to control the overall Type I error rate and avoid the inflation of false positives.
4. Data Exploration and Hypothesis Generation:
Before conducting formal statistical analyses, researchers should engage in exploratory data analysis to understand the distribution of variables, identify potential outliers, and generate hypotheses. This initial exploration helps guide subsequent analyses and ensures that statistical tests are appropriate for the data characteristics.
5. Confounding Variables and Adjustment:
Account for potential confounding variables that could influence the observed associations. Biomedical researchers need to carefully consider and control for confounding factors during statistical analyses to obtain more accurate and meaningful results. This may involve using statistical techniques such as multivariable regression or propensity score matching to control for confounding variables and isolate the effect of interest.
Navigate the challenges of using registry data and other public data, with expert guidance from a biostatistician. Check out Editage’s Statistical Analysis & Review Services.
Comments
You're looking to give wings to your academic career and publication journey. We like that!
Why don't we give you complete access! Create a free account and get unlimited access to all resources & a vibrant researcher community.
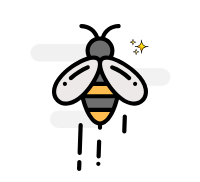
Subscribe to Conducting Research
Conducting research is the first and most exciting step in a researcher's journey. If you are currently in this stage of your publishing journey, subscribe & learn about best practices to sail through this stage and set yourself up for successful publication.