10 things biomedical researchers need to know when conducting simulation studies
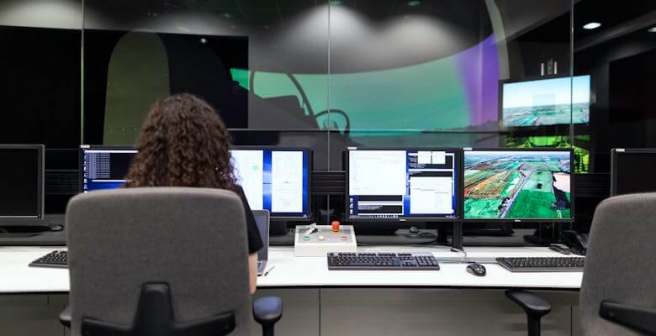
Simulation studies in biomedicine involve the use of computational models to mimic biological systems, processes, or interventions. These simulations enable researchers to explore complex biomedical phenomena, evaluate hypothetical scenarios, and make predictions about the behavior of biological systems under various conditions. Simulation studies can encompass a wide range of applications in biomedicine, including pharmacokinetic modeling, disease progression modeling, treatment optimization, and healthcare system simulation.
One of the primary advantages of simulation studies in biomedicine is their ability to provide insights into complex biological processes that may be difficult or unethical to study directly in human subjects. By using computational models, researchers can manipulate variables, simulate interventions, and observe outcomes in a controlled virtual environment. This allows for hypothesis testing, scenario exploration, and the generation of new scientific knowledge.
Additionally, simulation studies offer several practical advantages over traditional experimental approaches. They can be cost-effective, as they often require fewer resources and can be conducted entirely in silico. Simulations also allow researchers to study long-term or rare events that may be impractical to observe in real-time experiments. Furthermore, simulations can be used to explore the effects of interventions or treatment strategies without risking harm to patients.
Simulation studies also facilitate the optimization of biomedical interventions and healthcare delivery systems. By simulating different treatment protocols or healthcare policies, researchers can identify strategies that maximize efficacy, minimize adverse effects, or improve resource allocation.
Biomedical researchers conducting simulation studies should consider several key statistical aspects to ensure the validity and reliability of their findings. Here are some important considerations:
- Sample Size Determination: Ensure that the sample size is adequate to achieve sufficient statistical power. Power analysis should be conducted prior to the simulation study to determine the minimum sample size required to detect the effect size of interest with acceptable probability.
- Randomization and Replication: Incorporate randomization techniques to allocate subjects or experimental units to different simulation conditions or treatments. Replication of simulations is crucial to assess the consistency and reliability of results.
- Model Assumptions: Clearly define the underlying assumptions of the simulation model. Validate these assumptions to ensure they hold in the context of the biomedical application. Sensitivity analyses can be performed to assess the impact of violating assumptions.
- Validity and Generalizability: Evaluate the external validity and generalizability of simulation results. Consider whether the simulated scenarios accurately represent real-world biomedical phenomena and whether the findings can be extrapolated to broader populations or contexts.
- Bias and Confounding: Identify potential sources of bias and confounding in the simulation design. Implement strategies to minimize bias, such as blinding procedures and randomization techniques. Sensitivity analyses can help assess the robustness of results to different sources of bias.
- Measurement Error: Account for measurement error in the simulation study. Incorporate realistic error models and assess the impact of measurement uncertainty on study outcomes.
- Statistical Analysis Methods: Select appropriate statistical methods for analyzing simulation data. This may include descriptive statistics, hypothesis testing, regression analysis, survival analysis, or machine learning techniques, depending on the research questions and data characteristics.
- Simulation Output Analysis: Develop clear criteria for analyzing simulation output and interpreting results. Consider statistical summaries, graphical representations, and sensitivity analyses to explore the variability and uncertainty in simulation outcomes.
- Validation and Calibration: Validate the simulation model by comparing simulated results with empirical data or established benchmarks. Calibration techniques can be used to adjust model parameters to improve the fit between simulated and observed data.
- Reporting and Interpretation: Clearly document the simulation methodology, including model assumptions, parameter values, and analysis procedures. Transparent reporting facilitates reproducibility and allows readers to critically evaluate the study findings.
Conclusion
Simulation studies play a crucial role in advancing biomedical research by providing a powerful tool for hypothesis generation, experimentation, and decision-making in a wide range of biomedical domains. They offer a versatile and efficient approach to understanding complex biological systems and improving clinical practice. By addressing the above 10 statistical considerations, biomedical researchers can enhance the rigor, reliability, and interpretability of their simulation studies, ultimately advancing scientific knowledge and informing clinical practice.
Eager to delve into the fascinating world of simulation studies? Collaborate with an expert biostatistician under Editage’s Statistical Analysis & Review Services.
Comments
You're looking to give wings to your academic career and publication journey. We like that!
Why don't we give you complete access! Create a free account and get unlimited access to all resources & a vibrant researcher community.
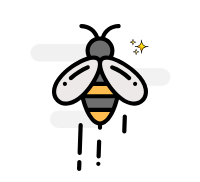
Subscribe to Conducting Research
Conducting research is the first and most exciting step in a researcher's journey. If you are currently in this stage of your publishing journey, subscribe & learn about best practices to sail through this stage and set yourself up for successful publication.