Does big data mean good data? 5 Challenges researchers face while handling big data sets


Big data
Big data has brought an unprecedented change in the way research is conducted. But what exactly does big data mean and why is it being considered as an additional paradigm to science? How can researchers make the most of big data in their work? Read on to know all about big data that you always wanted to know.
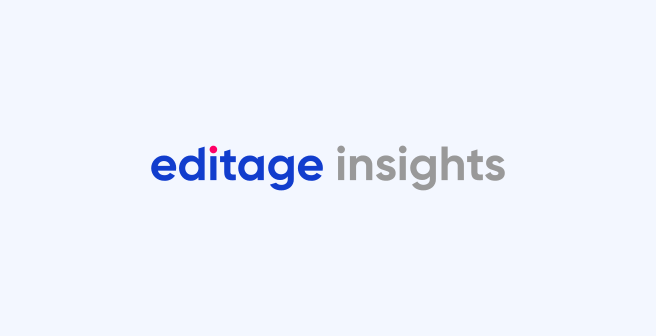
Big data has brought an unprecedented change in the way research is conducted in every scientific discipline. Where the tools for researchers were limited to the specificity of their fields, big data is now increasingly becoming a common tool across disciplines. The availability of big data sets and the capacity to store and share large volumes of data has opened several avenues of scientific exploration for researchers.
Being the foundation of research work, data is exceptionally valuable for researchers. Therefore, the data deluge is viewed as a boon by most researchers, particularly those working in the field of genetics, astronomy, and particle physics. While big data is now considered as an unparalleled paradigm of science, statisticians advice researchers to be wary of big data since the nature of big data is multidimensional and ever shifting. Researchers have embraced big data, but along with the opportunities it provides, it also brings complexities. Some of the major challenges academicians face while handling big data are:
1. Managing data effectively is tough: Storing large sets of data poses both infrastructural and economic problems for researchers who are not supported by institutions. Apart from this, curating and sharing large data sets is complicated since privacy, security, and integrity of data can lead to conflicting interests where international collaborations are involved. Therefore, there is a need for a sustainable economic model that will overcome infrastructural challenges and enable a smoother process for data-driven research.
2. Data collection gets prioritized over study design: Although data is vital for any study, at times, gathering data precedes in importance over a carefully designed study. Some researchers tend to harbor the misconception that more data directly relates to better research. Instead of focusing on the manner in which data is collected and the purpose of collecting it, large volumes of data are collected with the assumption that it would enhance the research. An example of this is a UK study which involved 20,000 children in order to assess the benefits of pasteurized milk. The study design and the scale at which the trial was conducted were criticized by William Gosset, a statistician. He said that due to inadequate randomization, a study with only 6 twin pairs would have been more reliable.
3. Analysis of big data requires special tools: Large volumes of data cannot be analyzed using conventional tools for data analysis. Standard software techniques are typically designed to analyze small sets of data. Big data, however, contains data of such magnitude that traditional tools can either take tremendous amount of time to analyze it or be unable to handle it. Therefore, special tools are required to connect data to models, enabling accurate evaluation of data. An example of this is Microsoft’s algorithm called FaST-LMM (Factored Spectrally Transformed Linear Mixed Model).
4. Data deluge can make data interpretation challenging: Big data contains data from various sources, making it multifaceted and difficult to interpret. For example, a data set containing information regarding world population would include data based on varied geographical locations, lifestyle, etc. and it may be collected using different techniques. Researchers may fail to consider all aspects of the data, resulting in incorrect conclusions. Hence, there is a need for developing reliable procedures of data interpretation that can overcome statistical biases.
5. The inclination to look for patterns in data is perilous: Since big data is large, researchers need to segregate useful data from the data sets. However, in most cases, instead of eliminating unrequired data, there is a tendency to look for patterns until a pre-conceived idea is supported by some evidence in the data. This is a dangerous pitfall when conducting research.
Data is undeniably a valuable asset—a fact corroborated by the declaration of data as a new class of economic asset by the 2012 World Economic Forum—and big data plays a seminal role in the advancement of science. However, the downsides of dealing with large volumes of data indicate that big data might not always spell good data. Therefore, researchers need to balance data with their subject-matter expertise and scientific reasoning to realize the optimum potential of big data.
To gain further insights into the challenges researchers face while collecting data and analyzing it, read the interview with Dr. Jo Roislien, a Norwegian mathematician, biostatistician, researcher in medicine, who holds a PhD in geostatistics and is a famous international science communicator.
Would you like a 1:1 consultation with an expert statistician? Check out Editage’s Statistical Analysis & Review Service.
Published on: Feb 06, 2015
Comments
You're looking to give wings to your academic career and publication journey. We like that!
Why don't we give you complete access! Create a free account and get unlimited access to all resources & a vibrant researcher community.
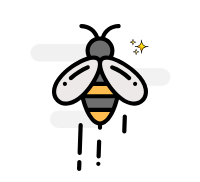
Subscribe to Conducting Research